Citizens of a modern democracy could enjoy a wide selection of independent media. Nevertheless, some news articles tend to contain biased content that could mislead its readers. More specifically, it has been shown that the pictures accompanying a news article strongly influence how readers perceive the topic [1]. Online news articles typically include an image at the beginning of the story being covered. This generally provides a context to the readers. This work set out to extract data from various Maltese newsrooms that might indicate instances of improper image use (image bias) in the corresponding online news articles.
Media-bias research in literature has not yet fully benefited from the recent advancements made in natural language processing (NLP) and computer vision (CV). Hence, this project proposes a data pipeline that would make use of a variety of machine learning models, as well as the state-of-the-art vision language pre-training (VLP) model, BLIP, which extracts key insights from the raw news article text that might indicate potential image bias.
The first step was to acquire news-article data by scraping the respective online newspapers from the web. Subsequently, techniques such as named-entity recognition (NER), keyword extraction, sentiment analysis, caption generation, text similarity, and image-text matching (ITM) were used to extract numerical metrics from individual news articles.
The ensuing results indicated clearly that ‒ during the time frame in which the newspapers were scraped ‒ not all the newspapers’ textual content aligned with the corresponding images to the same degree. These findings may hint at potential image bias within those newspapers that tended to stray below the average threshold. It is to be noted that one limitation of this research is that it is based on the assumption that the artificial intelligence models used were themselves free from bias.
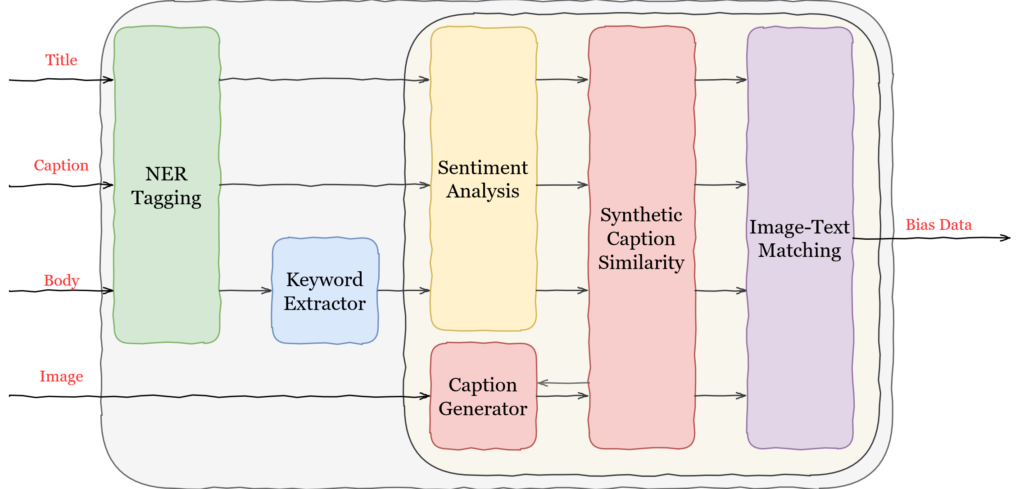
Figure1. The proposed data transformation pipeline
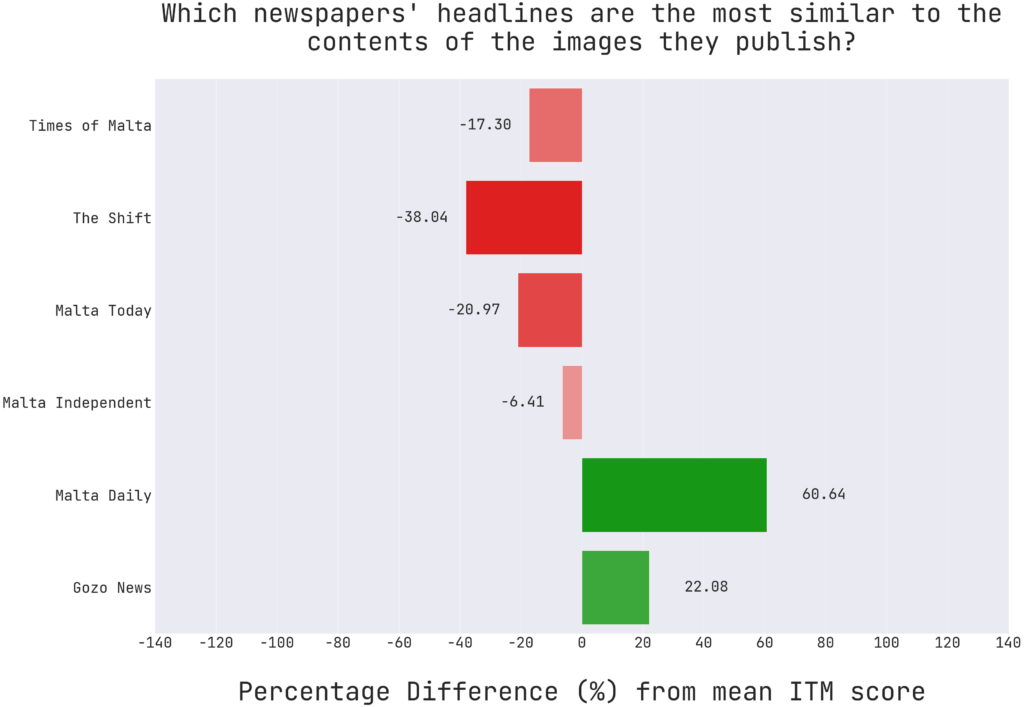
Figure 2. Bar chart displaying the findings of the study
Student: Gabriel Hili
Supervisor: Dr Dylan Seychell