The World Health Organisation (WHO) defines depression as being “characterised by a persistent sadness and a lack of interest or pleasure in previously rewarding or enjoyable activities”. Apart from displaying mental symptoms, such as demotivation and lack of concentration, depression could also trigger an array of physical symptoms. One of these physical symptoms, which is of interest to this study, is a change in speech, whereby persons suffering from depression might develop different speech characteristics when compared to non-depressed persons.
This study focused on analysing whether the above-mentioned characteristics could be identified by machine learning techniques, towards enabling the distinction between depressed and non-depressed speech.
The aim of this study was to research existing automatic depression detection (ADD) methods designed for depression detection and classification, as well as to propose architectural enhancements to these methods. Research into ADD is relatively recent, and currently offers very limited datasets in terms of amount and size. The chosen dataset for this study is the DAIC-WOZ dataset compiled by the University of Southern California. This contains audio material from interviews conducted between a virtual interviewer and a human participant. The study was based on a sample of 189 human participants with different levels of depression. The various levels of depression were calculated through a PHQ-8 questionnaire, which consists of eight questions concerning: interest in things, emotions, sleep levels, energy, appetite, self-confidence, concentration, and unusual behaviours in bodily movements.
To tackle this classification problem, two possible types of solutions were researched. These solutions included either the use of vocal biomarkers, such as the AVEC and GeMAPS feature sets, or making use of spectrograms. Examples of shallow machine learning models were trained using vocal biomarkers, while convolutional neural networks (CNNs) were trained using spectrogram arrays. From these researched approaches, it was decided to focus the proposed approach on CNNs, in view of their strong pattern-matching abilities.
Spectrograms represent the frequency-vs-time-information of audio signals and offer a breakdown of all the vocal behaviour of a speaker. An example of a spectrogram for a four-second audio clip could be seen in Figure 1. Besides showing message content, spectrograms also reveal useful information about the state of mind of the person speaking.
This study evaluated the effects of using both 1D and 2D CNNs using an ensemble averaging method to calculate the average accuracy score. Furthermore, the possibility of adopting a multiclassification approach to depression classification was duly tested. Instead of viewing mental health disorders as a binary problem (having / not having the disorder), a possible alternative approach would be to visualise mental conditions along a spectrum. By increasing the classification accuracy, a reduction of possible misdiagnosis of patients could be achieved.
ADD systems are not intended to supplant seeking help for mental disorders from medical professionals, but to provide a way with which to track progress and/or deterioration of mental health. This would ideally encourage individuals to seek help with any mental issues they might be experiencing, thus also helping reduce the negative stigma attached to mental issues.
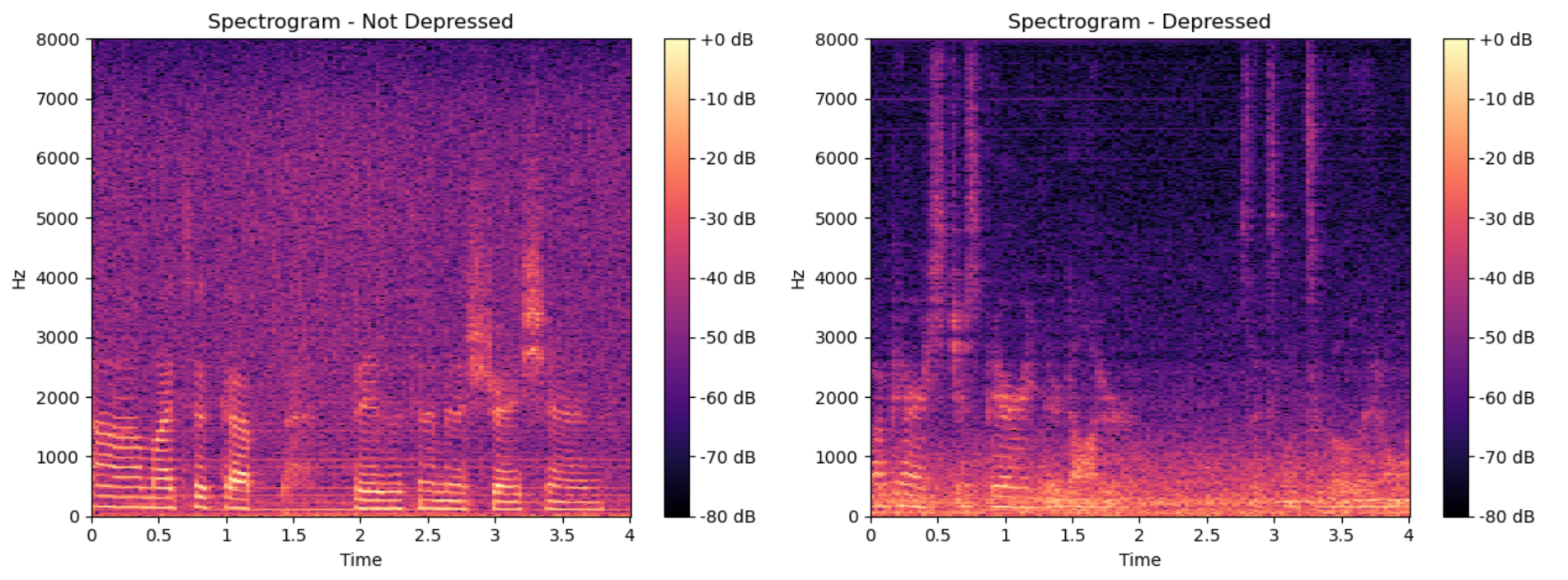
Course: B.Sc. IT (Hons.) Artificial Intelligence
Supervisor: Dr Andrea De Marco
Co-supervisor: Dr Claudia Borg