The problem of making predictions on the stock market has been extensively studied by market analysts, investors, as well as researchers from across different fields of study. This is due to the difficulty of the problem, and also to the potential financial gain that an efficient solution would bring about. . In view of the recent progress made in the field of machine learning, extensive research has been carried out on the issue of applying machine learning models to stock market predictions.
The efficient-market hypothesis (EMH) posits that the current asset price reflects any and all available information. This implies that any new information entering the stock market would be used, thus making it very difficult to predict future prices based on old prices. Due to this dynamic and the fluctuating nature of the stock market, certain underlying concepts start to change over time. This phenomenon is known as the concept drift. When concept drift occurs, the performance of machine learning models tends to suffer, sometimes drastically. This decline in performance occurs because the data distribution that was used to train the model is no longer in line with the current data distribution. The concept drift issue is not exclusive to the stock market, as it affects many real-world domains, such as weather prediction and sales forecasting. Concept drift has been noted in numerous occasions through the years. However, a popular example that appeared recently in mainstream media occurred in early 2021, when the GameStop (GME) stock, stopped following its ‘normal’ pattern and started to increase in price drastically.
The research presented in this study aims to answer the following research question: “Can a machine learning model that has been trained on a dataset containing previous stock prices, get better results if it undergoes a retraining process every time a concept drift has been detected?”. This question could be addressed by first examining and evaluating four concept drift detectors that, according to existing literature on the topic, have been proven to deliver good results.
Having selected the detector that promised the best results, the next step was to replicate research that used a state-of-the-art deep learning model to predict stock prices. The chosen concept drift detector was then attached to the replicated model. Each time a concept drift was detected, the model underwent one of several retraining methods. In the evaluation, the results of the basic model were compared with the results of the models that were fitted with a concept drift detector.
The conducted experiments highlight the effectiveness of each of the proposed retraining methods, as well as the extent to which each of the methods mitigates the negative effects of concept drift in different ways. The best observed result was a 2.5% increase in accuracy, when compared to the basic model.
While this research addresses the problem of concept drift in the stock market domain, the proposed techniques could potentially be used in other domains where concept drift is also a major issue., However, for this sort of generalisation to be applied, further experimentation would be required.
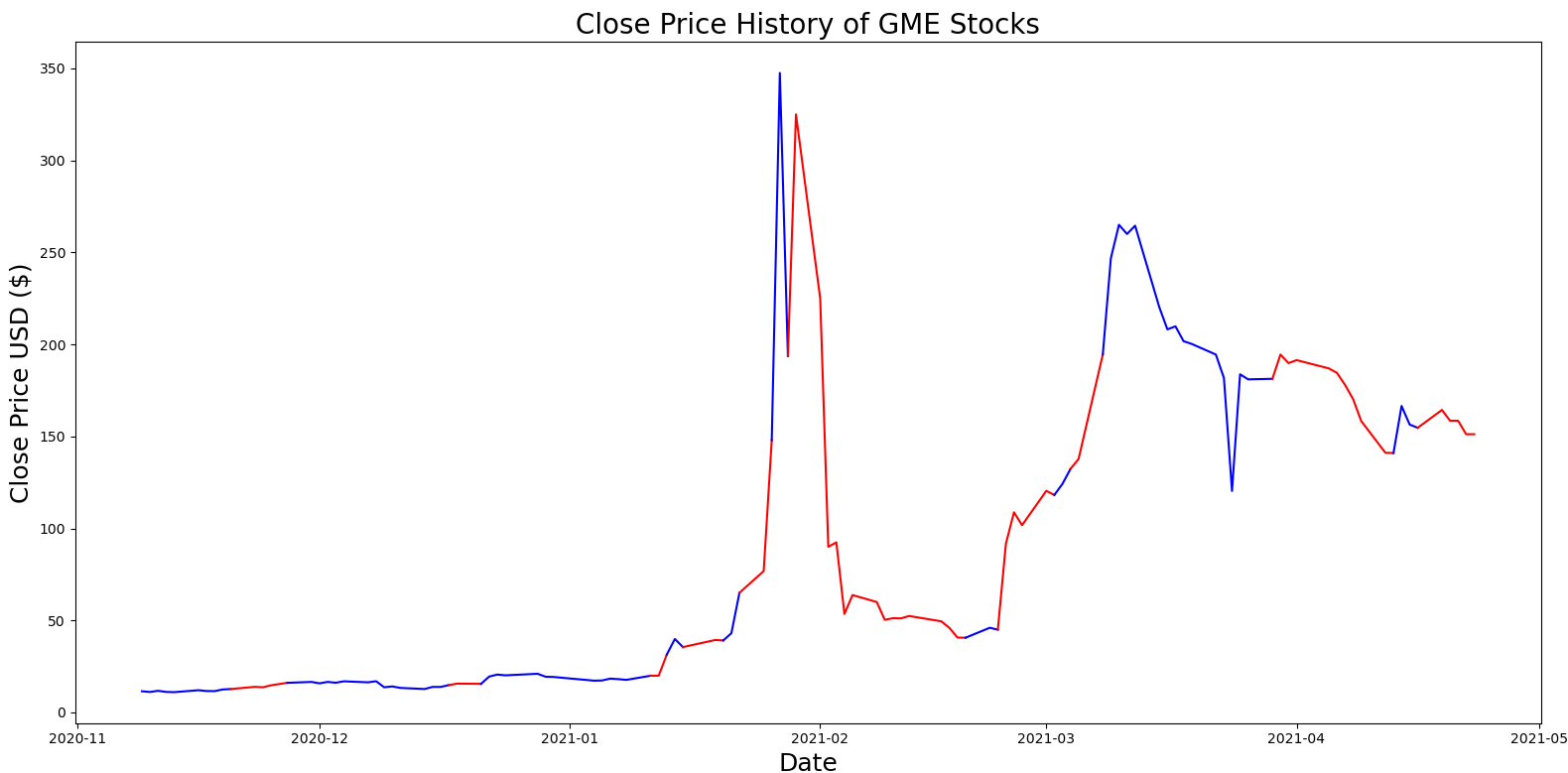
Course: B.Sc. IT (Hons.) Artificial Intelligence
Supervisor: Dr Charlie Abela
Co-supervisor: Dr Vincent Vella