In 2019, the EU registered just under 23,000 vehicle-related fatalities, with Malta losing 16 individuals to traffic accidents in that year. According to research, the majority of these fatalities could be linked to dangerous driving behaviour. Hence, in a bid to make drivers more aware of their driving behaviour, this research set out to investigate different machine learning algorithms, which could be used in conjunction with smartphone sensor data to detect fatal or near-fatal driving incidents.
Modern smartphones are equipped with a number of easily accessible sensors, such as an accelerometer, which measures the acceleration force applied to the device, and an orientation sensor that determines the rotation of the device. These sensors provide a better user experience, act as user input, or provide information about the external environment.
Aggressive manoeuvres, such as abrupt turning, braking, acceleration, or lane-changing are bound to be reflected in accelerometer readings on different axes. In the case of braking and accelerating, the value of the z-axis increases for an acceleration event, while it decreases for a deceleration event. The amount by which this value differs is linked to the aggressiveness of the manoeuvre. Similarly, turning events can be detected by variations in the values of the y-axis. These sensor values were captured in real-time through a developed smartphone application, which instructs the driver to perform such manoeuvres, and stores the appropriate data for future use. The stored accelerometer values for each axis are used to train multilabel machine learning algorithms, which classify the data to the different aggressive manoeuvres.
The developed application could also be used as a real-time driver evaluation tool. Securely mounted to the vehicle’s windscreen, the application would read the real-time acceleration data and use the trained model to detect aggressive events. The detected event would then be shown clearly, alerting the drivers of their behaviour.
The system proved to be capable of detecting events with a high accuracy, achieving over 98% accuracy on existent datasets with a recurrent neural network algorithm. The support-vector machine and random forest machine learning algorithms were also tested to solve the multilabel classification problem, achieving an accuracy of 96% and 93% respectively.
Apart from being used as a real-time indicator of one’s behaviour, leading to reduced road accidents, the transportation and logistics, and insurance sectors could also make use of such an application. In the insurance sector, new usage-based motor insurance schemes use one’s driving behaviour to set their insurance premiums. In the transportation and logistics sector, drivers could be monitored and rewarded for their good driving performance which – apart from reducing costs through a reduction of accidents, repair costs, vehicle downtime and insurance costs – would help increase safety of the road and the reduction of harmful emissions.
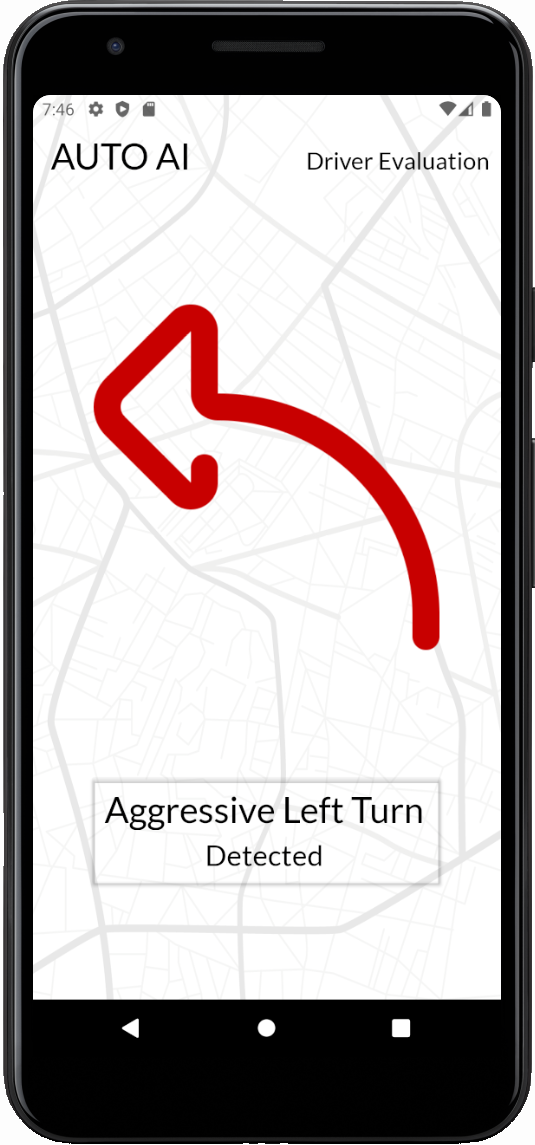
Course: B.Sc. IT (Hons.) Artificial Intelligence
Supervisor: Dr Josef Bajada