Ongoing research on Human Computer Interaction (HCI) is always progressing and the need for machines to detect human emotion continues to increase for the purpose of having more personalized systems which can intelligently act according to user emotion. When different languages portray emotions differently, this becomes a challenge in the field of automatic emotion recognition from speech. Different approaches may be adopted for emotion recognition from speech and in most cases systems approach the emotion recognition problem in a speaker-independent or context-free manner in order to supply models that work in different environments. These systems train on a number of diverse aspects including individuals’ speech, alternating between gender, age groups, accent regions or even single-word utterances.[1] Past works have been quite successful in this type of emotion recognition from speech, but in most cases testing was carried out using a mono-language corpus with one classifier and naturally obtaining a very high accuracy rate as shown in [2]. None of the current studies have as yet addressed the challenges of emotions being recognized in real-life scenarios, where one limited corpus will not suffice. We propose a system which takes a cross-corpus and multilingual approach to emotion recognition from speech in order to show the behaviour of results when compared to single monolingual corpus testing. We utilize four different classifiers: K-Nearest Neighbours (KNN), Support Vector Machines (SVM), Multi-Layer Perceptrons (MLP), Gaussian Mixture Models (GMM) along with two different feature sets including Mel-Frequency Cepstral Coefficients (MFCCs), and our own extracted prosodic feature set on three different emotional speech corpora containing several languages. We extracted a list of 8 statistical information values on our speech data: Pitch Range, Pitch Mean, Pitch Dynamics, Pitch Jitter, Intensity Range, Intensity Mean, Intensity Dynamics and Spectral Slope. The scope of the prosodic feature set extraction is to acquire a general feature set that works well across all languages and corpora. When presenting our results, we notice a drop in performance when unseen data is tested, but this improves when merged databases are present in the training data and when EMOVO is present in either training or testing. MFCCs work very well with GMMs on single corpus testing but our prosodic feature set works better in general on the rest of the classifiers. SVM obtains the best results both in cross- corpora testing and when cross-corpora testing is mixed with merged datasets. This concludes that SVMs are more suitable for unseen data while GMMs perform better when the testing data is similar to the training data. Although MLP were never the best performing machine model, it still performed relatively well when compared to an SVM. Meanwhile, KNN was always a bit less accurate on average than the rest of the classifiers. We evaluate all the obtained results in view of proving any elements that could possibly form a language-independent system, but for the time being results show otherwise.
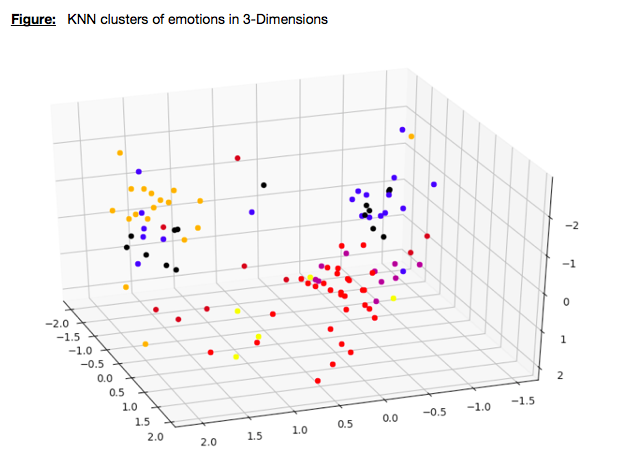
References
[1] J. Nicholson, K. Takahashi, and R. Nakatsu. Emotion recognition in speech using neural networks. Neural Computing & Applications, 9(4):290–296, Dec 2000.
[2] Yi-Lin Lin and Gang Wei. Speech emotion recognition based on hmm and svm. In 2005 international conference on machine learning and cybernetics, volume 8, pages 4898–4901. IEEE, 2005.
Student: Alessandro Sammut
Supervisor: Dr Vanessa Camilleri
Co-supervisor: Dr Andrea DeMarco
Course: B.Sc. IT (Hons.) Artificial Intelligence