In the world of motorsports, the ability to make strategic decisions is of paramount importance for determining the optimal outcome of a race, such as achieving the best possible finishing position. There are various factors to be taken into consideration when developing a strategic plan for a driver to follow during a race. Some of these factors include: track characteristics, weather conditions, and car set-up. However, one of the most crucial factors, or possibly the most, is the pit stop.
This study focuses on the Formula One (F1) racing series, where a pit stop is undertaken at least once, primarily to change tyres, serve a penalty, and/or to make adjustments or minor repairs to the car.
During a pit stop, the driver enters the pit at a reduced speed, resulting in loss of time. Additionally, the drivers lose more time as they stop in front of their garage to complete the pit stop. Hence, it would be imperative for the team to devise a clear and effective strategy to determine the optimal timing for pit stops and the appropriate tyre compounds to switch to.
Artificial intelligence (AI) has already been adopted in this sport, through practical applications in diverse domains such as car modelling, marketing and strategic decision-making, among others. Given the data-rich nature of F1, it is evident that AI algorithms play an increasingly important role in optimising performance. As mentioned above, AI has been adapted to automate strategic decision-making processes, namely concerning the pit stop.
This study attempted to build upon the virtual strategy engineer (VSE) model originally devised by Heilmeier et al. [1].This model utilises two artificial neural networks (ANNs) – one for pit stop decision-making and the other for tyre-compound selection. This model would allow for the optimisation of pit stop timings and the tyre compound selection for the race cars during a pit stop.
In this project, the effectiveness of the VSE’s strategy was evaluated through a number of tests, including a comparison with the real-life strategy employed by the drivers. The results demonstrated that the VSE effectively improved race outcomes by optimising strategies. Additionally, reinforcement learning (RL) algorithms were employed to further refine the decision-making process. By using the race simulation as an environment for the RL, it was observed that further improvements could be made to race outcomes, thereby enhancing strategic decision-making.
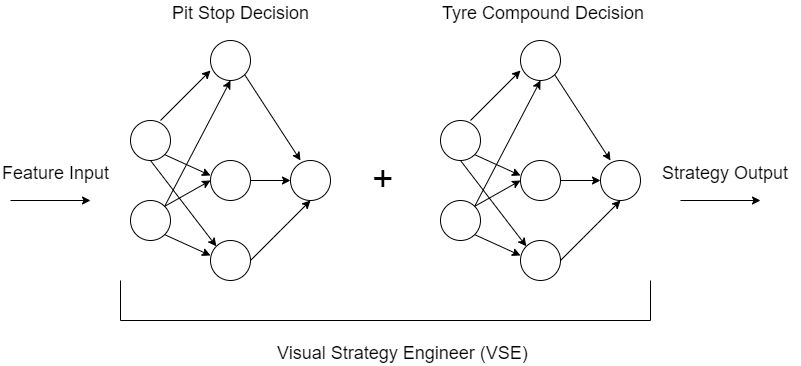
Figure 1. An overview of the virtual strategy engineer, as adapted from Heilmeier et al. [1]
Student: Charmaine Micallef
Supervisor : Dr Charlie Abela