Nuclear research for the purpose of physics and science is among the fastest-growing type in the world, yet a small scratch on the inside of a particle accelerator could jeopardise results. Now, partly in thanks to Master’s in Signal Process & Machine Learning student RYAN AGIUS, a cheaper and faster solution is being proposed.
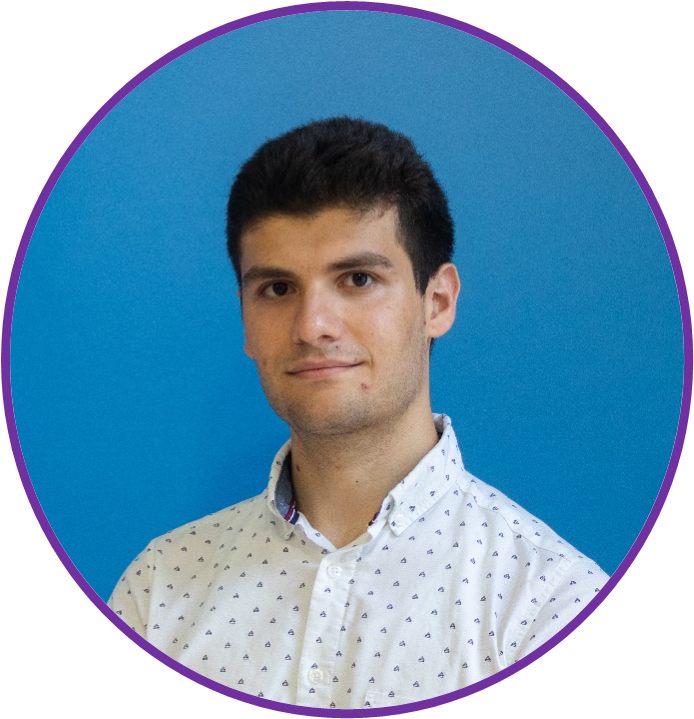
Nuclear research does not always have a nice ring to it: historical events have left many doubting the true benefits of unleashing the power of the atom. Yet, in reality, particle accelerators are helping physicists and scientists solve some of the universe’s most elusive questions with regards to the laws of nature and the laws of physics, as well as giving humanity the potential to have unlimited, carbon-free electricity.
Such research is done in tightly-controlled environments where scientists can safely smash charged particles (protons or electrons) together to analyse the way these react. For this process to occur, particle accelerators, which can come in the shape of a circular or linear collider, use a radiofrequency (RF) cavity.
These RF cavities are usually made from two copper shells welded together and coated with a layer of the metallic element, niobium. For them to work their magic, their temperature must be taken down to near zero kelvin degrees (equivalent to -273.15°C), as this gives them the perfect conducting qualities needed to accelerate particles. But there is just one issue with all this: the tiniest scratch or imperfection in the millimetre-thick niobium coating on the inside could mean the cavities just won’t work as well as they should, ultimately affecting the results of the experiment.
“One way scientists and engineers have been trying to find these imperfections is by cooling the cavity and seeing which parts of it heat up during operation, as these indicate the presence of defects,” Ryan explains. “But, this takes a lot of time, is quite expensive, and has to be repeated for each anomaly present on the surface as the process can only identify one at a time.”
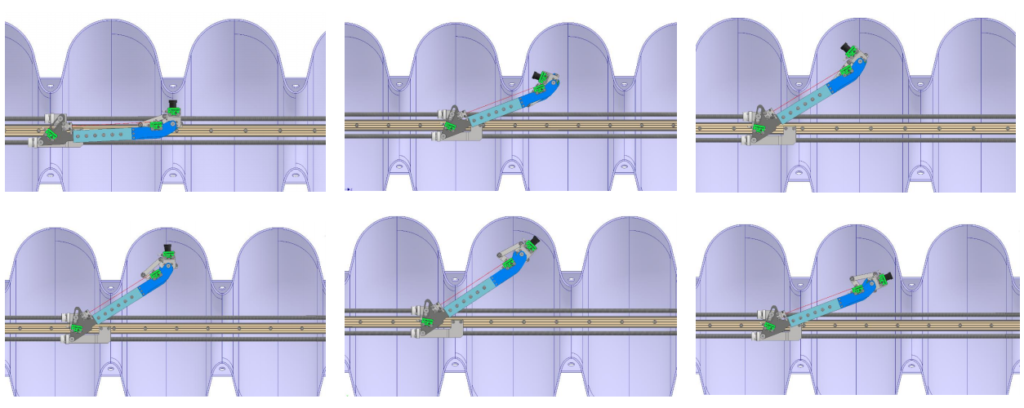
For the past year, Ryan has been part of an international team of students trying to help find a solution to this issue at CERN, the European Organization for Nuclear Research. The answer they have come up with is in the shape of a tiny camera that can be inserted in the RF cavity and which can then take over 50,000 images of the surface over the course of 50 hours. But this presented the team with some more problems, as the openings of these RF cavities are just 5cm in diameter, while the RF cavity’s design features numerous torus (doughnut) formations, which make scanning them for 10 micrometre (one millionth of a metre) defects using a camera on a metal arm quite a feat.
Ryan’s job in all this was two-fold: he first had to work on the optical system of the camera, and then create an algorithm that would be able to read those 50,000 images and come up with a report of whether there were any anomalies and where.
“The optics of the camera were indeed a headache, as normal lenses in such a small size did not offer us enough power to capture such small imperfections in the detail we require. But there was yet another issue: the robot arm could not ensure that the lenses we used were always at the same distance from the subject, meaning that not all photos had the same depth of field. This was all counteracted through the use of space-grade liquid lenses, which have a layer of oil that changes the shape of the lens when voltage is applied to it.”
Ryan then worked on the algorithm that could read the images and come back with a report. This algorithm had to understand what was an anomaly that needed to be fixed and what wasn’t. As Ryan explains, some scratches and the welding seam have no effect on how effective the RF cavity is. But how would the algorithm know what to include and not to include in the report, without the report coming back full of noise?
“In the end, it was decided that the wavelets (baby waves) mathematical concept would be used to better identify each anomaly from the photos. We combined this with another algorithm designed specifically to process images, from which we generated an accurate report that was able to identify anomalies that could significantly reduce the RF cavity’s performance.
“For the time being, however, we still have some issues with the algorithm recognising the very visually distinct welding seams as anomalies when they in fact do not affect the performance, but we are working closely with scientists from the Deutsches Elektronen-Synchrotron (DESY) nuclear research group to mitigate this problem and make the visual anomaly detector that much more viable.”
Ryan’s algorithm may now help bring this project a step closer to being used by CERN’s scientists, and may save them both time and money in their world-leading experiments.
“It’s been a fantastic journey,” Ryan, who was one of the first students to sign up to the Signal Process & Machine Learning Master’s, continues. “I had always wanted to do an AI course that had more practical rather than theoretical outcomes, and the fact that I got to work with the people at CERN just made the experience that much more memorable.”
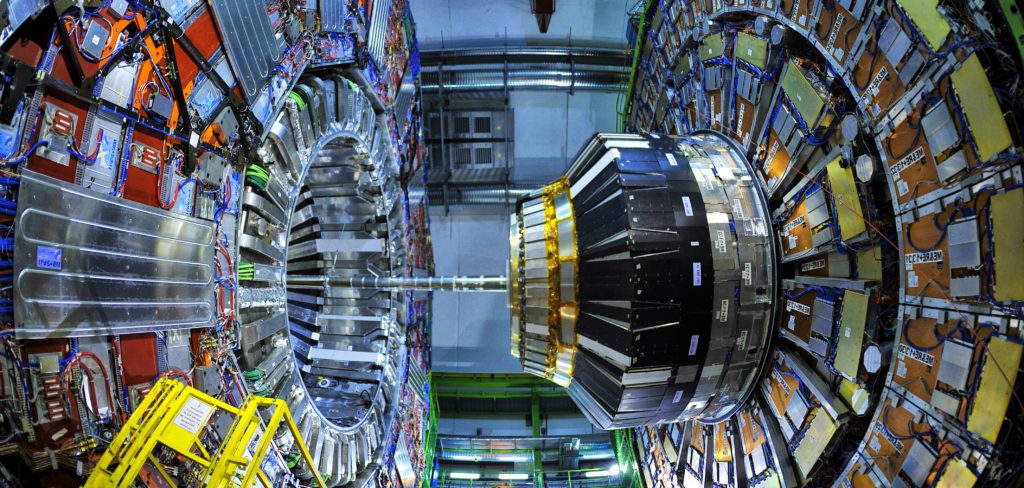