Gait analysis is the systematic study of human locomotion, employing observers’ eyes and brains, together with apparatus to measure body movements, body mechanics, and muscle activation. Analysing gaits is quite a tedious and generally lengthy procedure. Moreover, there is currently a very limited number of professionals equipped to undertake the analysis.
Typically, expensive and time-consuming marker-based technologies are employed in the procedure, in conjunction with several infrared cameras to generate kinematic data that would concisely quantify a person’s walking behaviour. This often discourages people from getting their gait examined.
This project sought to develop a mobile-based automated substitute to marker-based gait analysis, which would require less financial and computational resources. The proposed alternative could serve as a baseline for future implementations that would be accessible from an ordinary smartphone or web browser, and therefore cheaper and more user-friendly.
The flexibility of accessing gait analysis through a web application would encourage people to check their walking patterns more regularly, and if their issue were very severe, they could proceed to contacting a specialist in a timely manner.
In order that gait analysis could be made possible on a mobile device, a substantial amount of data was required. By collaborating with Mater Dei Hospital and the Chinese Academy of Sciences Institute of Automation (CASIA), a considerable amount of gait data was acquired. The data consisted of videos of people walking regularly or irregularly. Since the videos alone were not sufficient for the development of the proposed system, the videos were inputted into a pose estimator whose goal was to outline the skeleton of the person throughout the video. Additionally, the pose estimator was modified to record the coordinates of the main joints concerning a gait cycle (i.e,, hip, knee and ankle). These coordinates were then plotted as a scatter plot, where the gait cycle could be generated.
After the gait cycle of each video was extracted, the next step was to classify whether that gait cycle was either regular or irregular. This goal is achieved by passing the labelled gait cycles into a pattern- recognition architecture (model), which was then tested for accuracy with satisfactory results.
Finally, the classification of the gait cycle of a person was achieved through the upload of a video into the web application depicting that person walking. Correct classification was acquired by processing that video to extract the gait cycle and passing it on to the already trained model, which was set to output the nature of the gait cycles.
The application was tested out on persons having bad, good or slightly bad gaits to investigate the rigidity of the system. After a series of experiments, it could be concluded that the system performed with 94% accuracy.
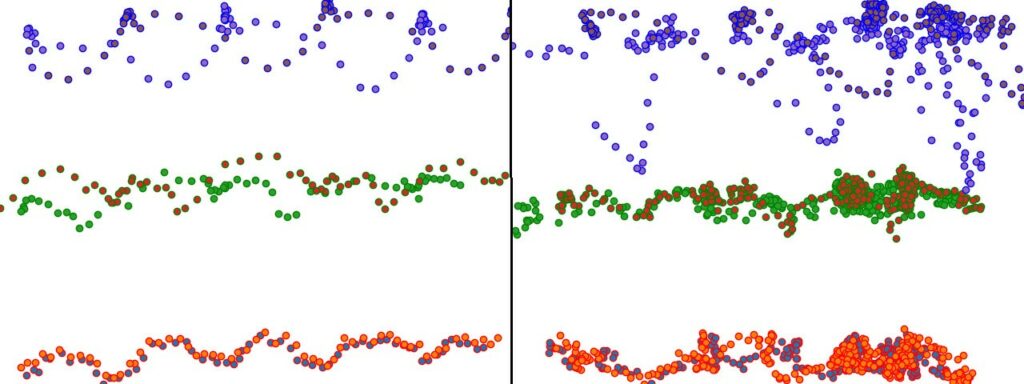
Student: Owen Agius
Course: B.Sc. IT (Hons.) Artificial Intelligence
Supervisor: Prof. Alexiei Dingli