PATRICK BEZZINA’s dissertation involved creating machine-learning algorithms that can generate correlated synthetic financial data. Here, he explains how this can help practitioners make better-informed choices and allow researchers to venture into new areas of research.
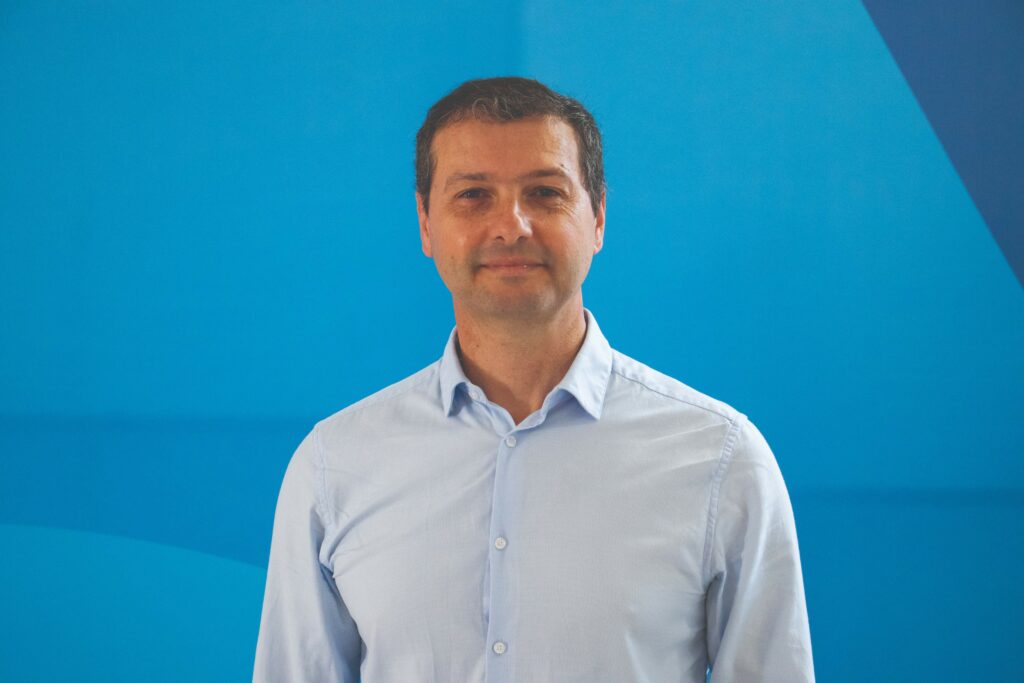
Financial data is the bedrock of any investment, particularly in an age when most trades happen electronically at speed. Yet, given the nature of the financial markets’ daily performance, collecting enough data points for large datasets to manage portfolios, requires practitioners to go back many years. Patrick Bezzina’s master’s in AI dissertation is looking to address that by applying time series generative models to generate synthetic, multi-asset, financial time-series data, as well as innovative way of infusing synthetic and real historic time-series data to improve portfolio performance.
“Financial market time-series data exhibits several properties that can make the modelling and prediction of financial markets extremely difficult. These include correlation; non-stationarity, meaning that the statistical properties of the data change over time; seasonality; fat tails, which is the occurrence of extreme events; and general market trends,” explains Patrick, who currently works as a Technology Consulting Senior Manager at the Malta office of the global assurance and consultancy firm, Ernst & Young.
As he continues, understanding these properties is important for analysing financial time-series data and making informed decisions. In addition, company news, financial results, macro-economic factors, and money markets can influence the valuation performance of financial assets from time to time.
“Considering that most markets operate an average of 250 days a year, there isn’t a lot of data per year, which affects the results of the research being undertaken by both practitioners and researchers when modelling the daily performance of the markets. In fact, one would need to go back 10 years just to get 2,500 data points and that’s assuming that this data is publicly available. This is generally not enough for tuning deep machine-learning models.”
A possible solution to this may be available in Patrick’s dissertation, which is testing generative deep, machine-learning models to study the distribution and correlational properties of market data and then create similar synthetic time-series data to augment real datasets. This, in turn, will help both researchers and practitioners seeking to better understand how different factors may impact the performance of a portfolio of assets.
In his study, Patrick employed TimeGAN, a deep generative adversarial framework that can generate synthetic time-series data with properties similar to the real-life version of the data available. In certain ways, it works similarly to ChatGPT, but instead of generating text, it generates time-series data samples.
The best part about TimeGAN, however, is that it comes with an interplay of five deep neural networks that work simultaneously to generate data and ensure that the said data mimics closely the real data by simultaneously learning to encode features, generate representations, and iterate across time.
“The project has been split into two experiments. In the first one, I used TimeGAN to create and compare market data features from commonly-used multi-stock datasets to see whether the synthetic data preserves the correlation structure. This was found to be the case, and both the synthetic and real data gave us practically the same information.
“Subsequently, the second experiment investigated whether utilising multi-asset synthetic data generated by TimeGAN in conjunction with real historic market data can generate better out-of-sample performance than a conventional, off-the-shelf portfolio optimisation method that only uses real data. The synthetically-generated price sequences were used in an adaptive de-risking mechanism whereby the assets that exhibited high risk at the end of the out-of-sample period were systematically filtered out from the portfolio.”
“The combined synthetic and real-life market data achieving around 46% higher cumulative portfolio returns”
The second experiment proved to be a resounding success, too, with the combined synthetic and real-life market data achieving around 46% higher cumulative portfolio returns, and an 18% higher annualised risk-adjusted performance Sharpe Ratio, when compared to the unfiltered portfolio.
Although the project is still in the research phase, the potential for it is huge. The encouraging results achieved by Patrick mean that this system could, in the future, help investment and fund managers optimise the mix of assets in their portfolios, make more intelligent investment decisions, and manage their risks better.
More than that, Patrick’s project continues to show that ICT can play a key role in many different areas. In fact, it was only after working for many years in the hedge fund industry, building sophisticated portfolio valuation and trade order management systems, that Patrick realised he could use the knowledge he had gained in capital markets to research AI, while simultaneously grow in his role. This can be true for many other sectors, and we are excited to see how else our students manage to combine ICT with their respective interests and expertise.