The demand for outpatient services is constantly on the increase. Catering for a high volume of patients inevitably causes the said services to encounter difficulties, such as clinic inefficiencies [1]. To mitigate this, the said services have undergone significant changes over the last 20 years [2]. Furthermore, pace of change quickens when both the patients and medical staff make use of the relevant technology to better address the fluctuating rates of referrals from general practitioners (GP) that tends to exhaust specialist resources [1].
This study consists of analysing clinical processes, designing dashboards and developing a solution by applying prediction algorithms. Clinical-appointment scheduling processes have been designed in business-process model notation (BPMN) to better understand how appointments and referrals are performed in the clinic. Business intelligence (BI) dashboards were designed to represent such information in an effective format, and to facilitate clinic analysis. Furthermore, prediction algorithms were applied to the dataset to generate the likelihood of a patient missing an appointment, thus triggering effective future appointment scheduling and helping to prevent missed reschedule opportunities [5, 6, 7].
A case study that has been chosen for the proof-of- concept attempts to address appointment scheduling problems within the Urology Clinic at the Outpatients Department at Mater Dei Hospital. These concern the appointments that are often assigned to non-urgent cases, and are usually not scheduled according to criteria such as patient appointment urgency or age of patient age, among others. Additionally, certain non-urgent, follow-up appointments could be postponed for the benefit of the truly urgent cases. The prediction algorithms enable clinicians to plan for future appointments by knowing which appointments are likely to be missed, and consequently to reschedule the urgent cases accordingly. Moreover, dashboards portraying valuable information would assist the staff in understanding the state of the clinic and patient throughput details [3, 4]. Such patient demographics could aid the consultant and other medical staff to set criteria for incoming appointments resulting from GP referrals.
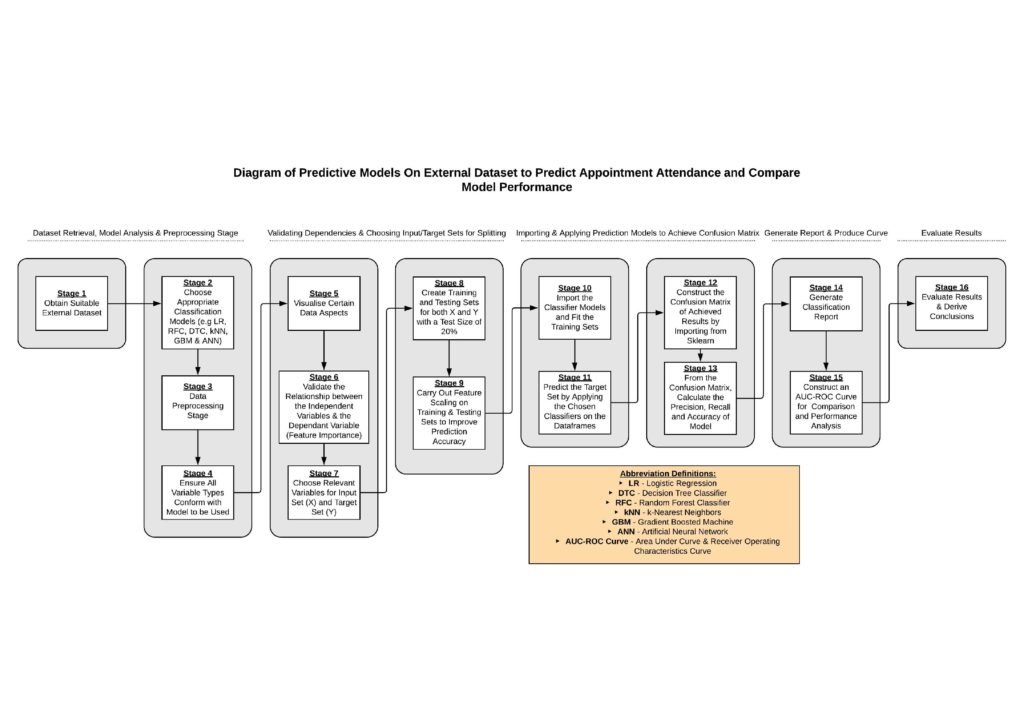
The requisite data for the project, including information regarding requirements at the clinic, were obtained by organising meetings with the consultant and other key medical professionals working at the clinic. An interview was set up with the clinic consultant to discuss in detail clinical processes, current issues and key performance indicators that would best be visualised in dashboards. A usability study was conducted with five participants to gather their feedback regarding the dashboards, their functionality and design. Upon reviewing the available feedback, it was noted that the various respondents were in favour of such dashboards, as they found them to be of significant assistance to medical staff. Since the usability score was very high, it was concluded that the dashboards were suitably designed for this scenario.
At the time of the study, the clinical patient administration system (CPAS) under review, did not capture the required amount of clinical data. Hence, an external dataset had to be sought for the purpose of testing the prediction models. The methodology for applying prediction models on the dataset was divided into stages, as shown in Figure 1. The performance of these prediction models yielded very satisfactory results. The ANN model appeared to be best suited to function on unknown data, and hence could be considered as a possible solution for managing appointment allocation at the Outpatients Department. The outcome of this study could have been more realistic and applicable, had the CPAS captured more data with regard to clinic appointments, schedules and GP referrals.
References/Bibliography:
[1] E. Winpenny, C. Miani, E. Pitchforth, S. King and M. Roland, “Improving the effectiveness and efficiency of outpatient services: a scoping review of interventions at the primary– secondary care interface”, Journal of Health Services Research & Policy, vol. 22, no. 1, pp. 53-64, 2016. Available: 10.1177/1355819616648982.
[2] Babiker A, El Husseini ME, Al Nemri A, Al Frayh A, Al Juryyan N, Faki MO, Assiri A, Al Saadi M, Al Zamil F., “Health care professional development: Working as a team to improve patient care.” Sudan J Paedi atr 2014; 14(2):9-16. Available: https://www.ncbi.nlm.nih. gov/ pmc/articles/PMC4949805/pdf/sjp-14-9.pdf.
[3] S. Buttigieg, A. Pace and C. Rathert, “Hospital performance dashboards: a literature review”, Journal of Health Organization and Management, vol. 31, no. 3, pp. 385-406, 2017. Available: 10.1108/jhom-04-2017-0088.
[4] K. Lee et al., “A novel concept for integrating and delivering health information using a comprehensive digital dashboard: An analysis of healthcare professionals’ intention to adopt a new system and the trend of its real usage”, International Journal of Medical Informatics, vol. 97, pp. 98-108, 2017. Available: 10.1016/j.ijmedinf.2016.10.001.
[5] D. Hanauer and Y. Huang, “Patient No-Show Predictive Model Development using Multiple Data Sources for an Effective Overbooking Approach”, Applied Clinical Informatics, vol. 05, no. 03, pp. 836-860, 2014. Available: 10.4338/aci-2014-04-ra-0026.
[6] C. Elvira, A. Ochoa, J. Gonzálvez and F. Mochón, “Machine-Learning-Based No Show Prediction in Outpatient Visits”, International Journal of Interactive Multimedia and Artificial Intelligence, vol. 4, no. 7, p. 29, 2017. Available: 10.9781/ijimai.2017.03.004.
[7] I. Mohammadi, H. Wu, A. Turkcan, T. Toscos and B. Doebbeling, “Data Analytics and Modeling for Appointment No- show in Community Health Centers”, Journal of Primary Care & Community Health, vol. 9, pp. 1-5, 2018. Available: 10.1177/2150132718811692.
Student: Redent Zammit
Course: B.Sc. IT (Hons.) Computing and Business
Supervisor: Dr. Conrad Attard