The sheer popularity of football means that most matches are extensively covered before, as well as after, the match. One particular practice that most news outlets follow is that of writing a brief, post-game report on the main highlights occurring throughout the match, such as goals scored or controversial decisions taken by the referee. Needless to say, such a task tends to be time-consuming, as it requires the writer to watch the match and then write the report. Moreover, online portals seek to produce and upload the report in as little time as possible from the end of the match, while the interest is still at its peak.
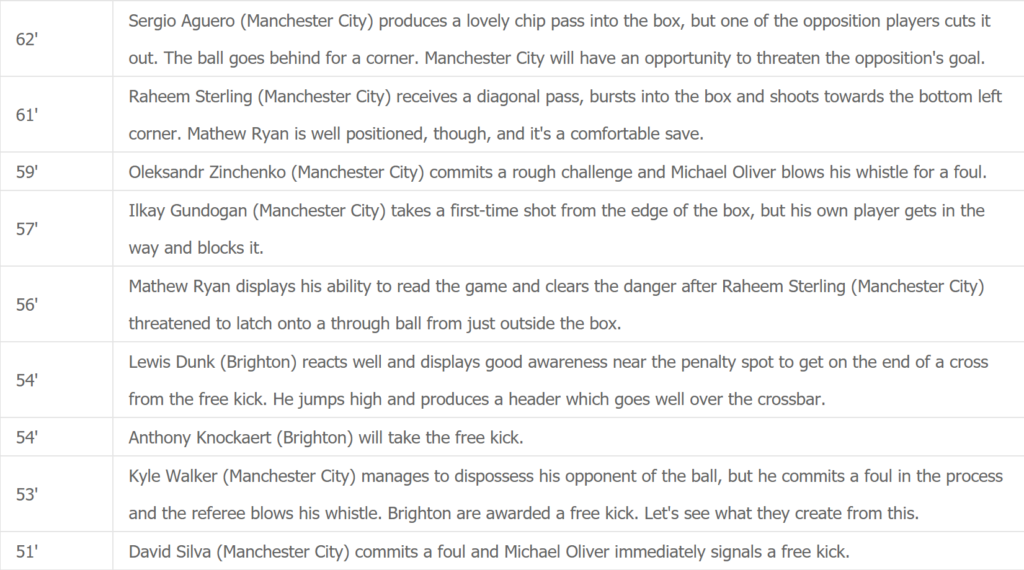
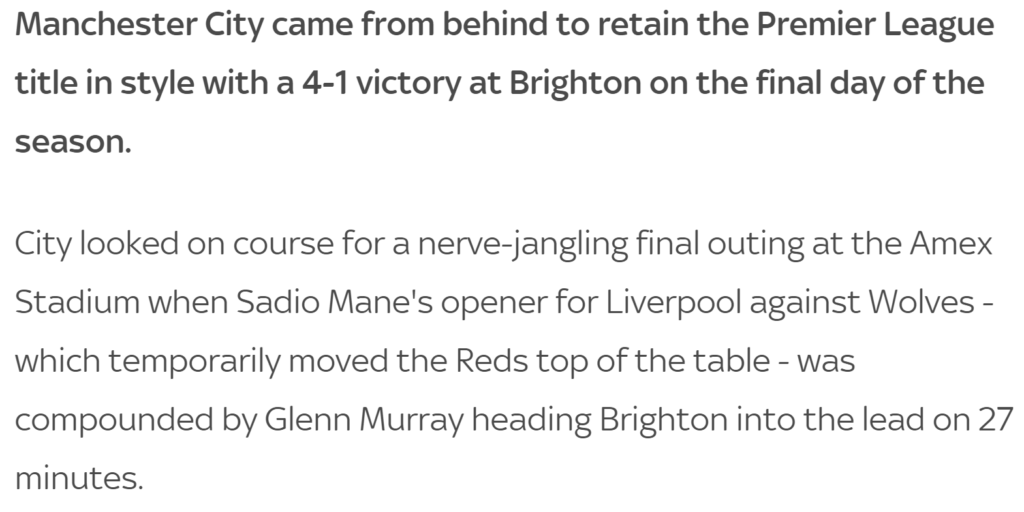
The main aim of this research was to propose a suitable architecture to automatically generate a football- match report from the commentary of any given match. The problem was framed as a special kind of extractive summarisation, whereby each sentence in the commentary was considered as a candidate for inclusion in the final report. From each ‘candidate sentence’, a number of features were extracted so that they could be scored and ordered.
The features employed for this study include those found in typical summarisation tasks, such as the position of a candidate sentence, as well as its sum of TF.IDF weights. A number of domain specific features, such as the inclusion of explicit highlight markers, were also utilised. These features are mainly based upon others used in similar research. After the commentary, the sentences were organised in the best combination of sentences, according to a set word count, and were subsequently selected to compile the final report. The study has taken into account that certain events occurring during a given football match tend to occur frequently together and in an ordered manner. This research has defined such phenomena as episodes and recognises them using sequential rule mining. The study also proposes a secondary architecture, which also accounts for episodes when compiling the final report. The performance of this data pipeline is compared and contrasted with one that does not consider episodes. Finally, the performance of both pipelines was measured against a number of task- specific and general summarisation baselines to evaluate their validity in addressing the issue under discussion.
Student: Jake Seracino
Course: B.Sc. IT (Hons.) Artificial Intelligence
Supervisor: Dr. Joel Azzopardi
Co-supervisor: Mr. Nicholas Mamo