A computed tomography (CT) scan provides detailed cross-sectional images of the human body using X-rays. With the increased use of medical CT, concerns were expressed on the total radiation dose to the patient.
In light of the potential risk of X-ray radiation to patients, low-dose CT has recently attracted great interest in the medical-imaging field. The current principle in CT dose reduction is ALARA (which stands for ‘as low as reasonably achievable’). This could be achieved by reducing the X-ray flux through decreasing the operating current and shortening the exposure time of an X-ray tube.
The higher the dose of X-rays within a specific range, the higher the image quality of the CT image. However, a greater intensity of X-rays could potentially cause more bodily harm to the patients. Conversely, using a lower dose of radiation can reduce safety risks however this would introduce more image noise, bringing more challenges to the radiologist’s later diagnosis. In this context, low-dose CT image-denoising algorithms were proposed in a number of studies towards solving this dilemma.
Although there are many models available, the task of low-dose CT image denoising has not been fully achieved. Current models face problems such as over-smoothed results and loss of detailed information. Consequently, the quality of low-dose CT images after denoising is still an important problem.
This work has sought to improve upon existing models and discover new models that could solve the low-dose denoising problem. A high-level architecture of the system is shown in Figure 1. The trained model produces denoised CT images from low-dose images, as shown in Figure 2. The models were tested at different dose levels on a custom-developed dataset obtained from Mater Dei Hospital. The best model from the tested machine learning techniques was chosen on the basis of image quality and the model’s efficiency.
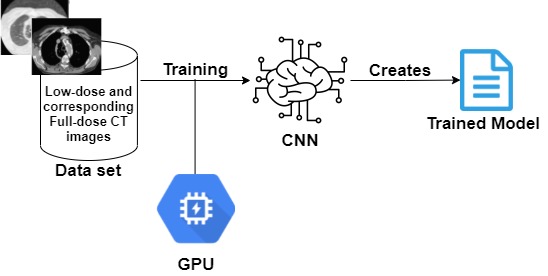
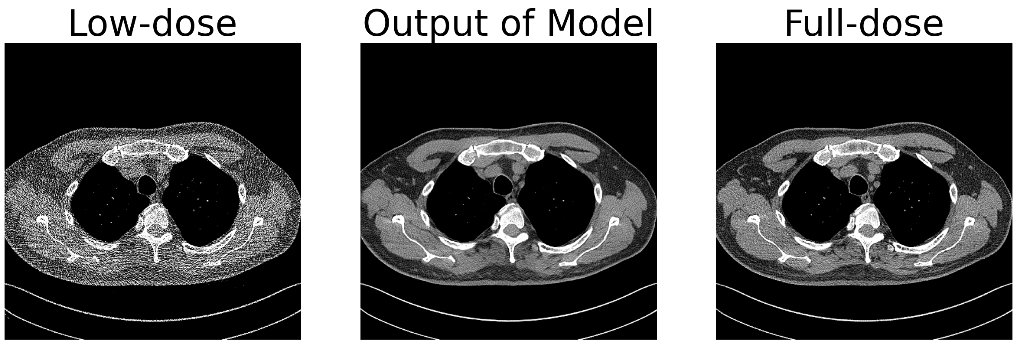
Course: B.Sc. (Hons.) Computing Science
Supervisor: Prof. Ing. Carl Debono
Co-supervisor: Dr Francis Zarb and Dr Paul Bezzina