Alzheimer’s disease (AD) is a neurological disease that often results in dementia. AD causes patients to experience severe cognitive decline, and also leads to an inability to perform basic bodily functions, such as walking or swallowing. Eventually, the condition becomes fatal. Currently, there is no treatment but an early diagnosis would facilitate the management of the disease. Thus, the patient may also be eligible for medication that could help reduce visible symptoms, possibly also slowing down the degenerative process.
In the case of AD patients, structural irregularities of the brain could be identified through neuroimaging techniques, such as magnetic resonance imaging (MRI). Neurologists examine the brain scans obtained through such techniques, and combine the analysis with the patient’s medical history and various clinical tests to provide a diagnosis of the disease.
In recent years, multiple computer-aided diagnosis (CAD) techniques have been proposed to provide an automated diagnosis of the disease, using brain scans from neuroimaging techniques. A number of studies in the field have applied the deep learning (DL) approach towards enhancing AD diagnosis, with promising results.
This study set out to investigate the use of different DL models trained on a dataset of MRI scans, in order to effectively distinguish between AD patients and healthy persons. A CAD system was implemented, combining the use of different preprocessing techniques to clean the raw MRI scans to be inputted in the DL models. These, in turn, were trained to provide an automated diagnosis of the disease.
The DL models proposed in this study have been duly evaluated in order to identify the best performing models. This exercise suggested that the proposed system could achieve promising results for the automated diagnosis of AD.
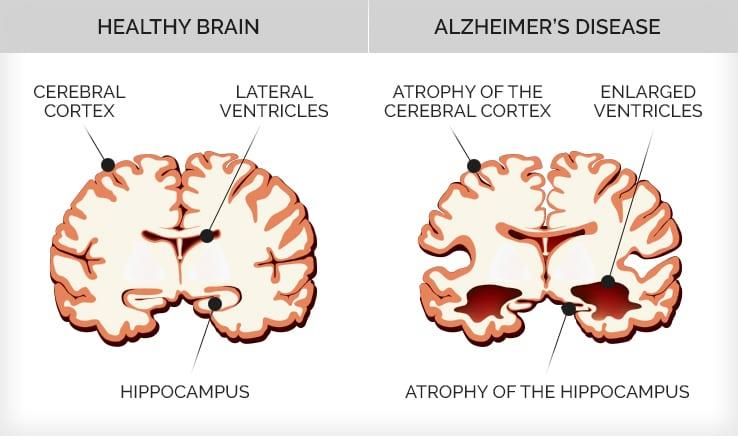
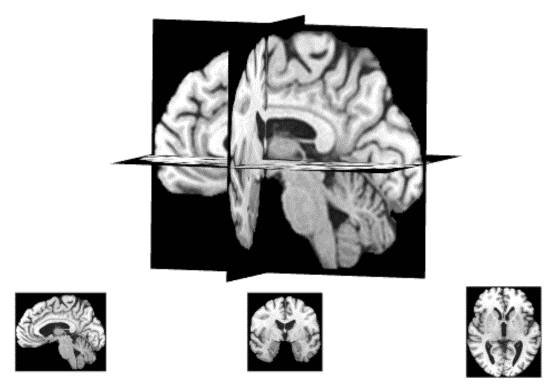
Course: B.Sc. IT (Hons.) Software Development
Supervisor: Joseph Bonello
Co-supervisor: John Abela