Link prediction has become a ubiquitous presence in online social networks (OSNs). A prominent example is the ‘People You May Know’ feature on Facebook. In information-oriented OSNs, such as Twitter, users choose whom to follow, on the basis of a number of factors. Although personality is one of the primary determiners influencing our social relationships, its impact within OSNs is often overlooked.
Personality could be represented by the ‘Big Five’ model, which identifies five dimensions: Openness to Experience, Conscientiousness, Extraversion, Agreeableness, and Neuroticism. It has been found that explicitly surveying users for these traits is not a viable option. However, by employing the relationship between language and personality, as reported by Mairesse et al [1], a user’s personality could be recognised without the need to resort to user surveys.
This research focused on extracting personality traits from textual content, and observing how these would relate to the established links between OSN users. In order to achieve this, we considered state-of-the-art research and developed personality recognition and link prediction components.
A basic representation of the personality recognition component is offered in Figure 1. Different approaches were investigated in line with existing research, comparing a variety of machine learning models and optimisation techniques. These models were trained on datasets containing users’ micro-blog postings and their Big Five trait values. The best performing models managed to recognise personality from text alone, achieving results that are comparable with the state-of-the-art.
The effect of user personality towards a real-life Twitter network was studied by applying the above-mentioned personality recogniser. Although correlations between the personality of followers and followees were found, it was also observed that users had their own implicit personality preferences in terms of who they follow. An example is outlined in Figure 2. The Personality-Aware Link Prediction Boosting (PALP-Boost) component takes these preferences into account and improves accuracy across various topological-based link-prediction algorithms, highlighting the utility of the underlying psychological attributes within OSNs.
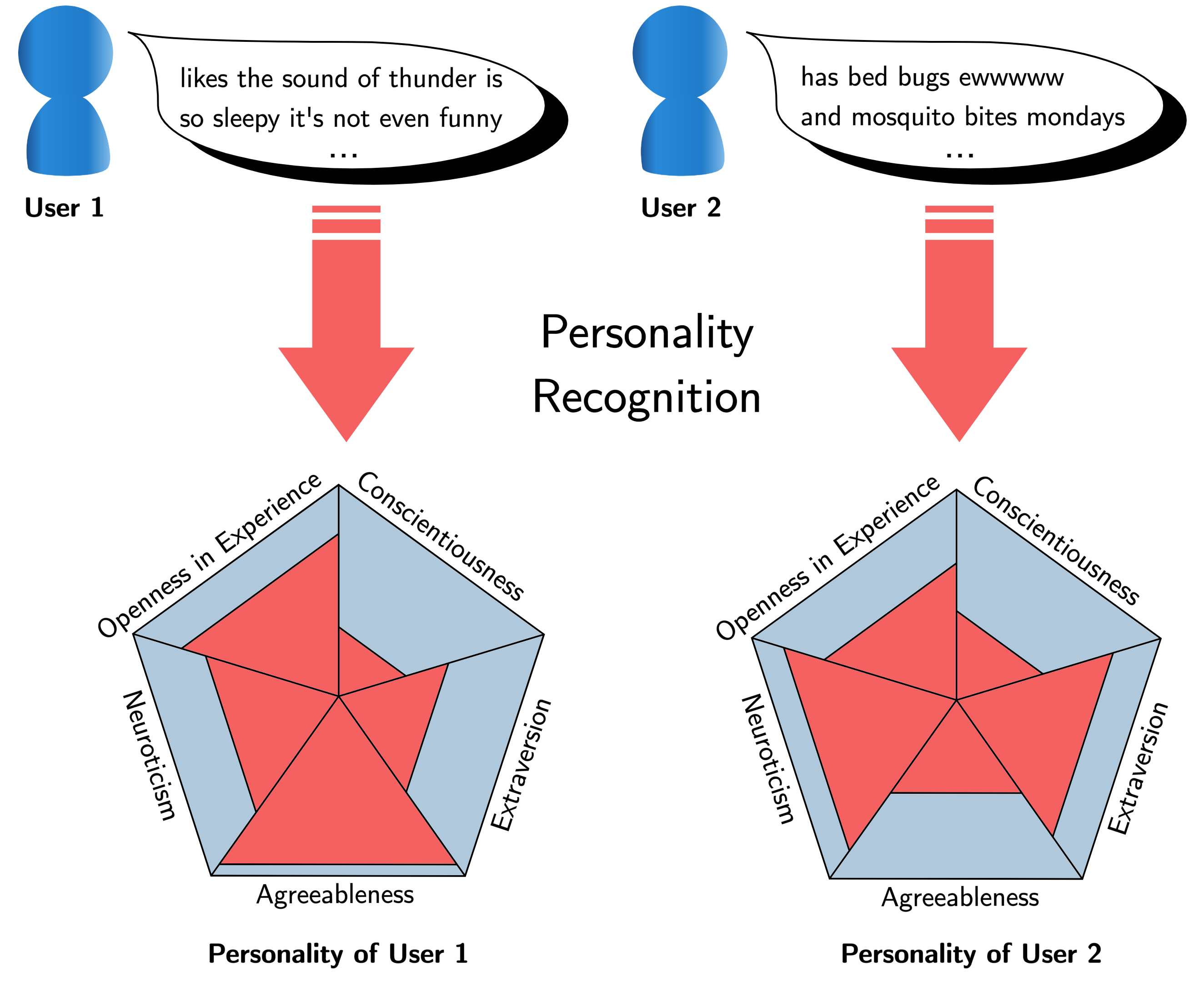
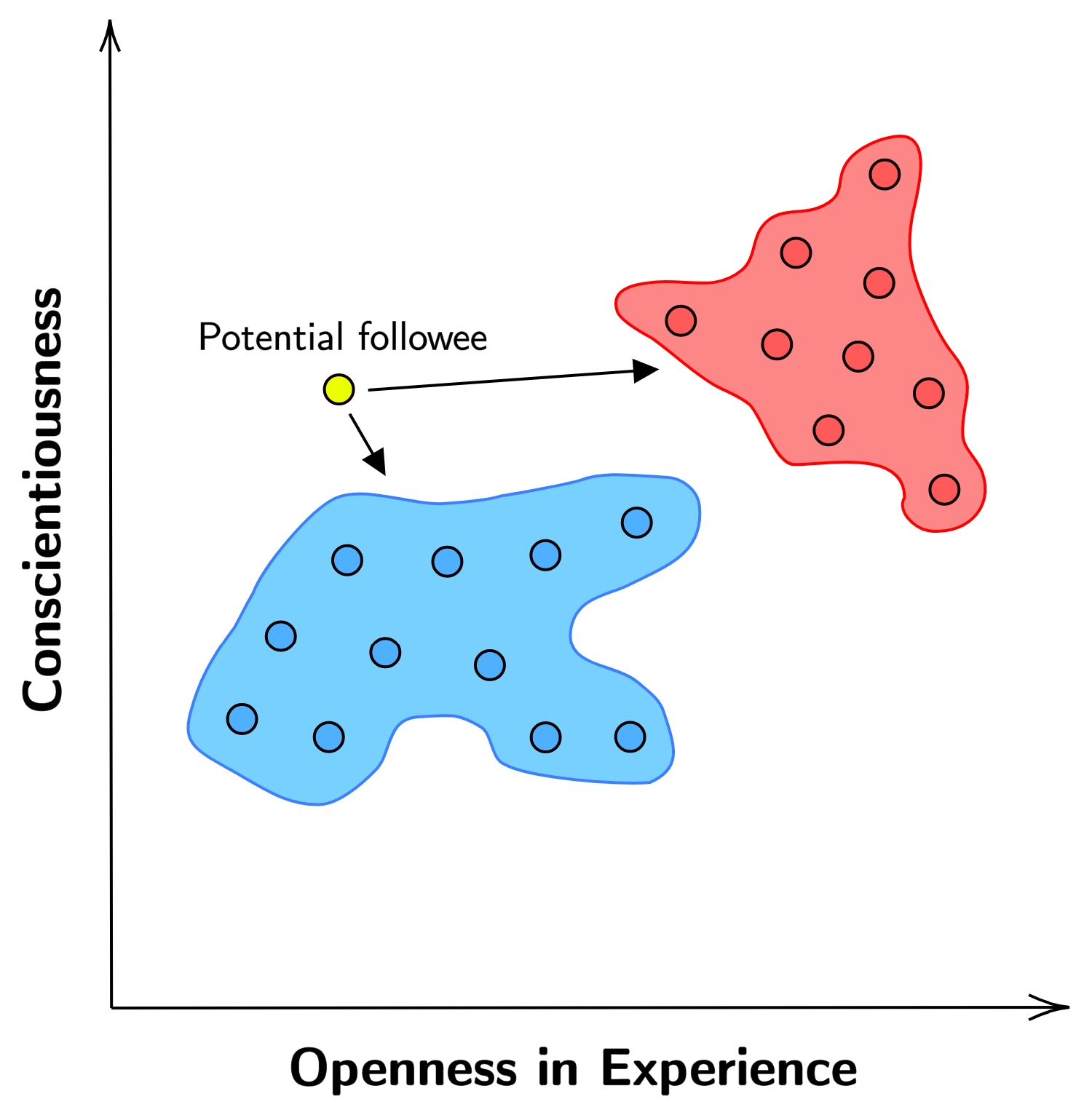
References/Bibliography
[1] Mairesse, F., Walker, M., Mehl, M. and Moore, R., 2007. Using Linguistic Cues for the Automatic Recognition of Personality in Conversation and Text. Journal of Artificial Intelligence Research, 30, pp.457-500.
Course: B.Sc. IT (Hons.) Artificial Intelligence
Supervisor: Dr Charlie Abela