Electroencephalography (EEG) is a biomedical technology that measures brain activity. Apart from being used to detect brain abnormalities, it has the potential to be used for other purposes, such as understanding the emotional state of individuals. This is typically done using expensive, medical-grade devices, thus being virtually inaccessible to the general public. Furthermore, since most of the currently available studies only work retrospectively, they tend to be unable to detect the person’s emotion in real time. The aim of this research is to determine whether it might be possible to perform reliable EEG-based emotion recognition (EEG-ER) in real time and using low-cost prosumer-grade devices such as the EMOTIV Insight 5.
Figure 1. The EMOTIV Insight 5 Channel Mobile Brainwear device
This study uses a rolling time window of a few seconds of the EEG signal, rather than minutes-long recordings, making the approach work in real time. The information loss between the number of channels of a medical-grade device and those of a prosumer device is also analysed. Since research has shown that different persons experience emotions differently, the study also analysed the difference between generic subject-independent models and subject-dependent models, fine-tuned to each specific subject.
Different machine learning techniques, such as support-vector machines (SVM) and 3D convolution neural networks (3D-CNN) were used. These models were trained on classifying 4 emotions ‒ happy, sad, angry, relaxed ‒ utilising the dataset for emotion analysis using physiological signals (DEAP). The best models achieved a 67% accuracy on the subject-independent case, rising to 87% for subject-dependent models, in real-time using only 5 channels. These results compare well with the state-of-the-art benchmarks that use the full medical-grade 32-channel data, demonstrating that real-time EEG-ER is feasible using lower-cost devices, making such applications more accessible.
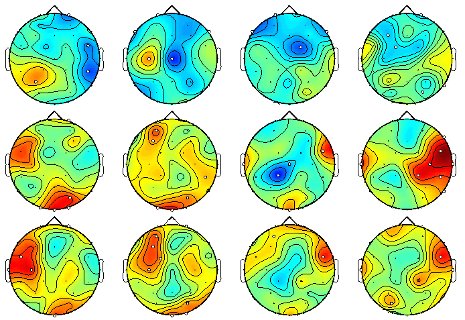
Figure 2. Brain activity of individuals experiencing one or more emotions
Course: B.Sc. IT (Hons.) Artificial Intelligence
Supervisor: Dr Josef Bajada