Product placement or embedded marketing involves the process of finding strategic locations for particular objects within a scene, in such a way that one’s attention would shift to the designated product. The use of advanced artificial intelligence and computer vision is expanding into every area of our lives. Recently, there has been rapid development in deep learning, which helped bring about a number of breakthroughs in computer vision.
One of the aforementioned breakthroughs is visual saliency, which is the branch of computer vision that generates attention-based models of a given scene using the features mentioned in the previous paragraph. Through human evolution, certain characteristics of an image, such as colour and contrast, make an object stand out from its neighbours and attract one’s attention. These are most of the time characterised by regions of different contrast, different intensity values and even orientation of features.
This dissertation evaluates how the use of visual-saliency techniques could improve product placement analysis by implementing an objective product-ranking system. This system was developed to observe a scene with a number of products, and then seek to predict upon which one the customer’s gaze would turn first. The system initially uses a state-of-the-art object detector to detect the products in the scene while another component ranks the detected product, using a technique based on a saliency segment-ranking algorithm. A final component was also included in order that, should there be multiple instances of the same product in a scene (e.g., a supermarket shelf) the system would detect and remove the product from the ranking. The modular aspect of this system allows many aspects of the product-ranking technique to be altered, such as substituting the saliency map-generating algorithm, which would allow evaluating the effectiveness of the different configurations and which components would most affect the accuracy of the rankings.
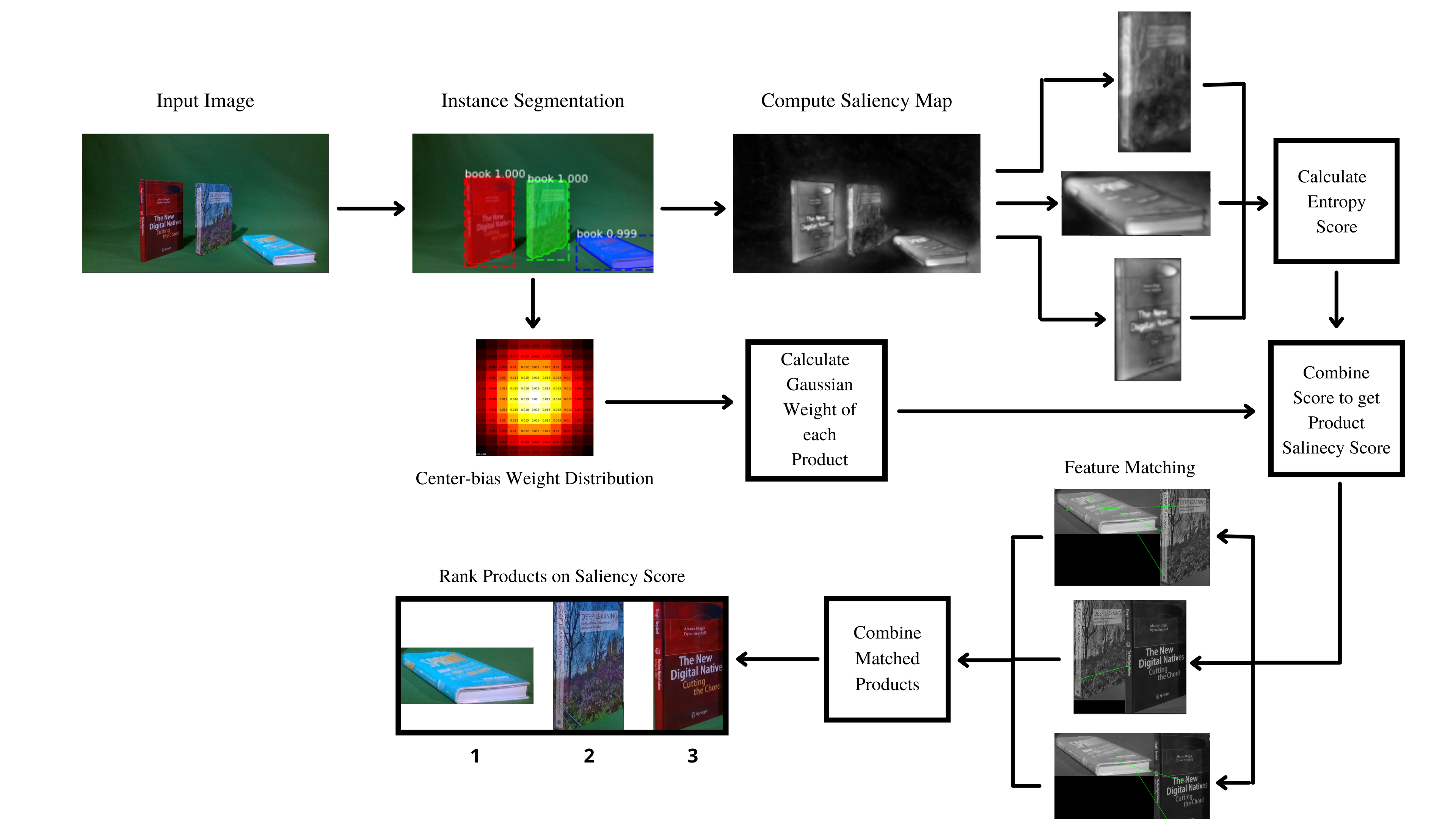
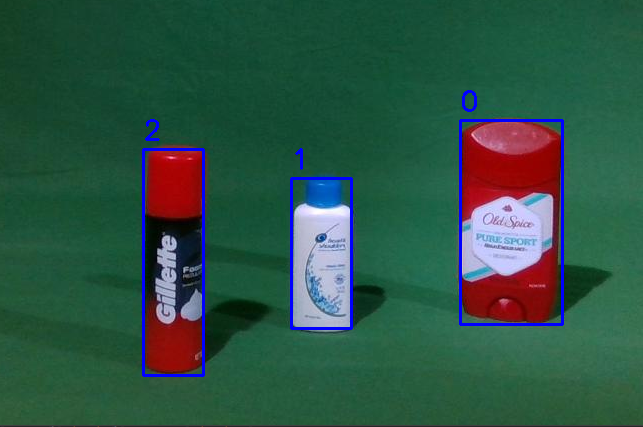
References/Bibliography
D. Seychell and C. J. Debono, “An Approach for Objective Quality Assessment of Image Inpainting Results,” 2020 IEEE 20th Mediterranean Electrotechnical Conference ( MELECON), 2020, pp. 226-231
D. Seychell and C. J. Debono, “Ranking Regions of Visual Saliency in RGB-D Content,” 2018 International Conference on 3D Immersion (IC3D), 2018
Course: B.Sc. IT (Hons.) Artificial Intelligence
Supervisor: Mr Dylan Seychell
Co-supervisor: Prof. Ing. Carl Debono