With the increasing sophistication and accessibility of deepfake-generation techniques, as well as the widespread sharing of fake news concerning political figures, there is a pressing demand for automated systems that could detect fake content. This demand was the main motivation for this study, which focused on deepfake-detection techniques that could accurately identify fake video media.
The selected approach was to create a deep learning (DL) model based on the research of Pandiyarajan [3], which employs error level analysis (ELA) for identifying suspicious areas in an image. This study made use of a dataset of frames from actual and deepfake videos featuring various politicians. The deepfake videos were generated using one of the major software programs for creating deepfakes, DeepFaceLab [2]. However, this did not exclude using other resources, such as Faceswap [1] which is also an open-source software for creating deepfake videos. This was done in order to demonstrate the ease of producing such fake media and level of accessibility of such media. The dataset consisted of 1190 authentic and 1678 fake images in JPG format, and a label to mark the fake images.
In addition to the above, pre-processing steps were taken, such as applying ELA, which is a method that determines whether an image has been tampered with, as well as image normalisation, which adjusts the range of pixel intensity values. The evaluation results confirmed that the experiments demonstrated by the CNN models by implementing the ELA techniques on the constructed dataset could detect deepfake images with a higher average than normal images. In addition, the study provides an insight into transfer learning techniques for pre-trained ImageNets (i.e., pre-trained convolution neural networks used for image classification), which were also applied to the created dataset. All models made use of the Adam optimiser, which is an extended version of the stochastic gradient descent optimiser algorithm that has an exponential learning rate of 1e-3.
Proposing a digital-forensics investigation system for detecting deepfakes involving political figures, this study contributes to the collective knowledge of combating misinformation and fake propaganda. The findings have implications for media verification and fact-checking organisations, and the proposed approach could be integrated into existing digital-forensics technologies to improve their effectiveness in identifying fake media.
Overall, this study emphasises the necessity of technological innovation and interdisciplinary collaboration in combating increasingly complex forms of digital fraud, and safeguarding the credibility of public debate.
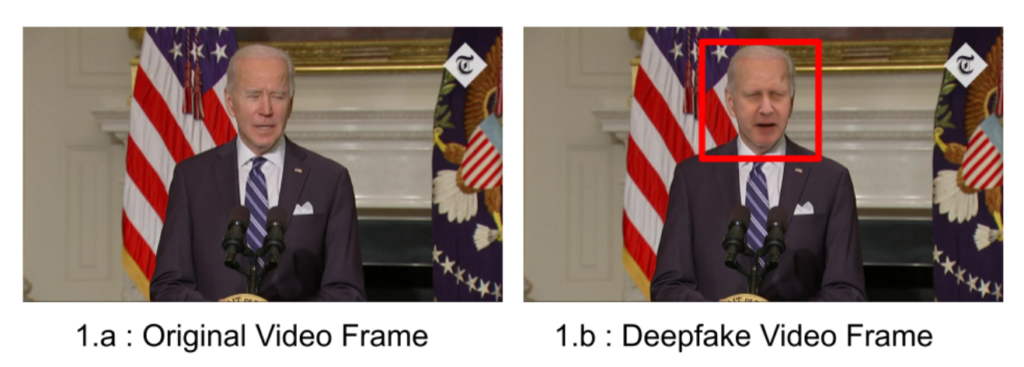
Figure 1. Genuine frame compared to a deepfake frame
Student: Adriana Ebejer
Supervisor: Dr Joseph Vella