The motivation behind this project was to contribute towards market penetration of wind energy and help increase its competitiveness vis-à-vis fossil fuels, with the ultimate aim of mitigating the negative effects of climate change.
To date, the major challenge hindering the reliance on wind energy is its unpredictability for power generation, due to its inherent dependence on wind ‒ this being a highly volatile resource, among other reasons. This unreliability threatens the performance of the electricity grid, rendering wind energy a less trustworthy option when compared to other sources and, hence, decreasing its market value.
This work sought to address the unreliability of wind energy through the setup and training of artificial intelligence (AI) models in order to predict the power generated from a wind turbine depending on current and forecasted weather conditions. This would help bring about accurate power scheduling and maximum exploitation of wind energy.
The project focused on forecast horizons of 6, 12 and 24 hours, as these were deemed to be the most important horizons for the deployment of wind energy in the electricity grid. The forecasts that were created produced a prediction of the average power generated for each hour within the given time horizon. Each forecast was created using multiple AI models, with the aim of identifying the best method for each use case. The dataset used to train the models contained weather data and wind farm data, such as the power that was generated and current wind speed.
Once the training was completed, the trained models were then evaluated to determine which model would be the best for each use case. To evaluate their accuracy, multiple forecasts were created using each model for each time horizon across all seasons, to verify their effectiveness over a variety of weather conditions.
The best-performing models provided highly accurate forecasts, with minimal training time, confirming the viability and potential of AI-powered forecasts for wind energy.
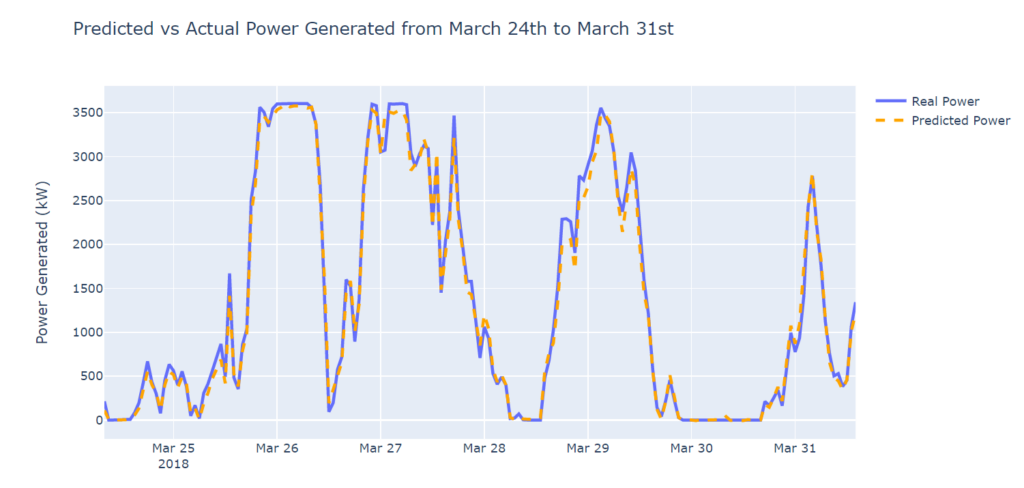
Figure 1. Predicted vs actual power for 24 – 31 March 2018
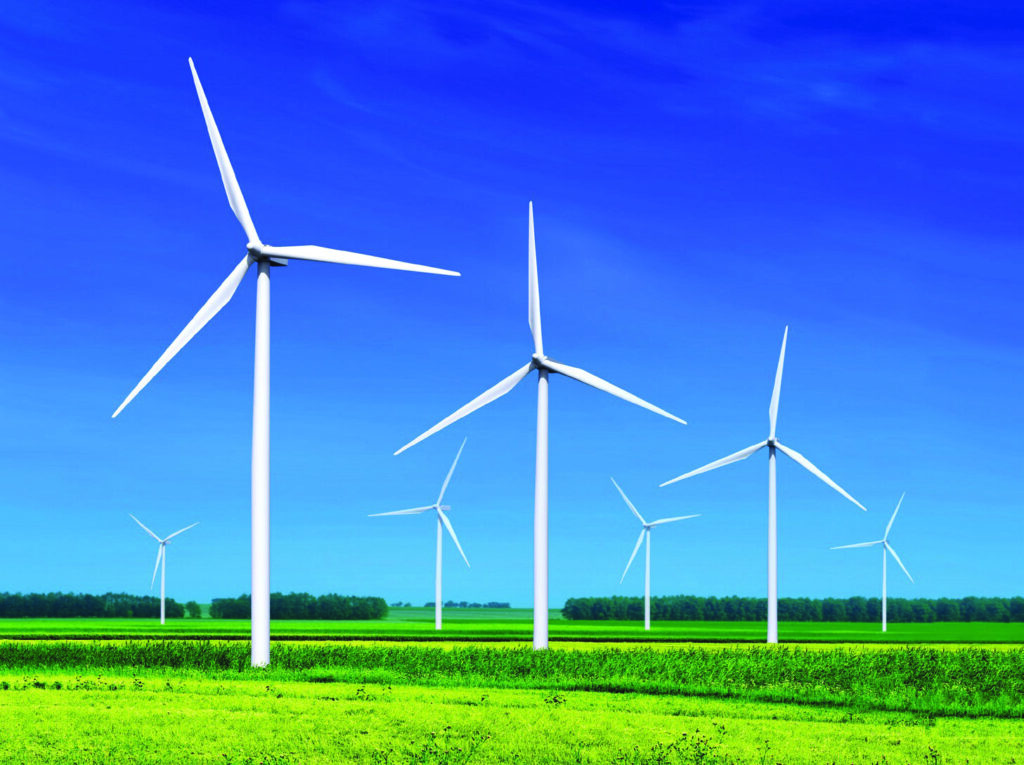
Figure 2. Wind turbines
Student: Ryan Mifsud
Supervisor: Dr Joel Azzopardi