In today’s world, artificial intelligence (AI) has been well integrated into various industries, not least in the media. News platforms continuously publish new news content every day. Hence, it is essential to offer personalised suggestions to individual users, be they new or regular visitors.
The algorithms behind the recommendations often remain opaque, leading to concerns about trustworthiness and potential biases. As the demand for transparency in AI-driven recommender systems grows, it becomes increasingly important to develop techniques that could explain the rationale behind the recommendations provided by the systems.
Current recommendation systems use various techniques to offer personalised news content. Although these methods have proven to be effective in providing personalised recommendations, they tend to lack the capacity to provide a straightforward explanation for the recommendations they produce. As a result, the users may not fully understand the logic behind the recommendations and would not be able to validate their quality or level of reliability.
The aim of this study was to enhance the transparency and interpretability of the recommendation process by merging similarity-based recommendation algorithms with interpretable machine learning. By utilising natural language processing, the system would produce textual explanations, visual representations and customised similarity scores to determine the importance of the suggested articles. This would result in a more comprehensive and accountable system.
The proposed techniques seek to address bias concerns in the news recommendation system by shedding light on the factors influencing the recommendations. Whilst providing clear and concise explanations for the recommendations made, the system would help generate trust among users.
Apart from enhancing the transparency of the system, an assessment of user comprehension was included in the study in order to establish how well the users could understand the explanations provided. This not only allowed analysing the impact of the explainability features but also made it possible to identify the effectiveness and importance of the system’s explanations to users interacting with the system.
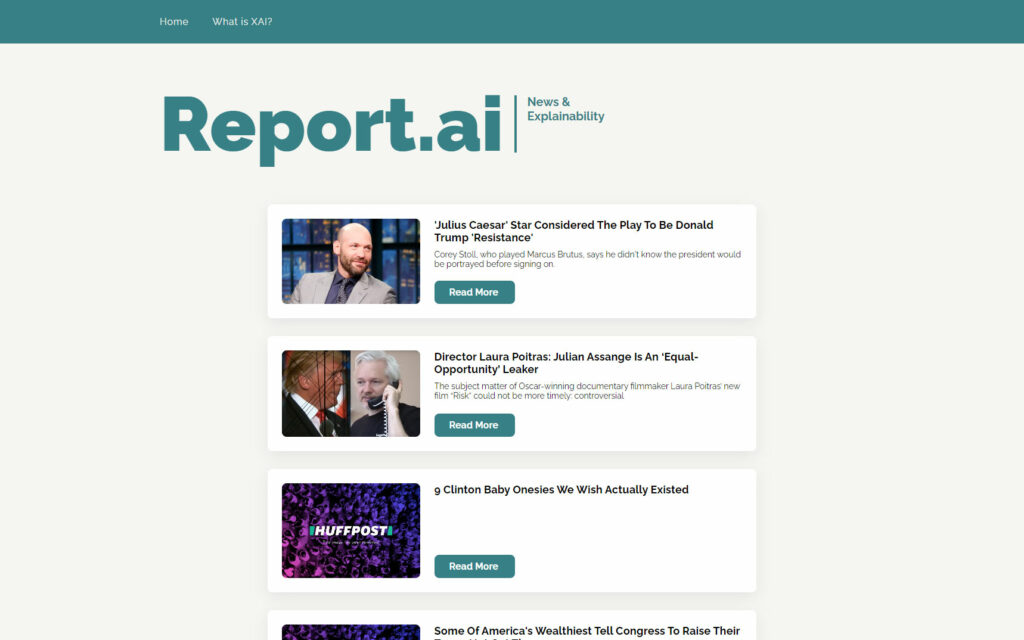
Figure 1. The home screen visible to the user upon opening the app
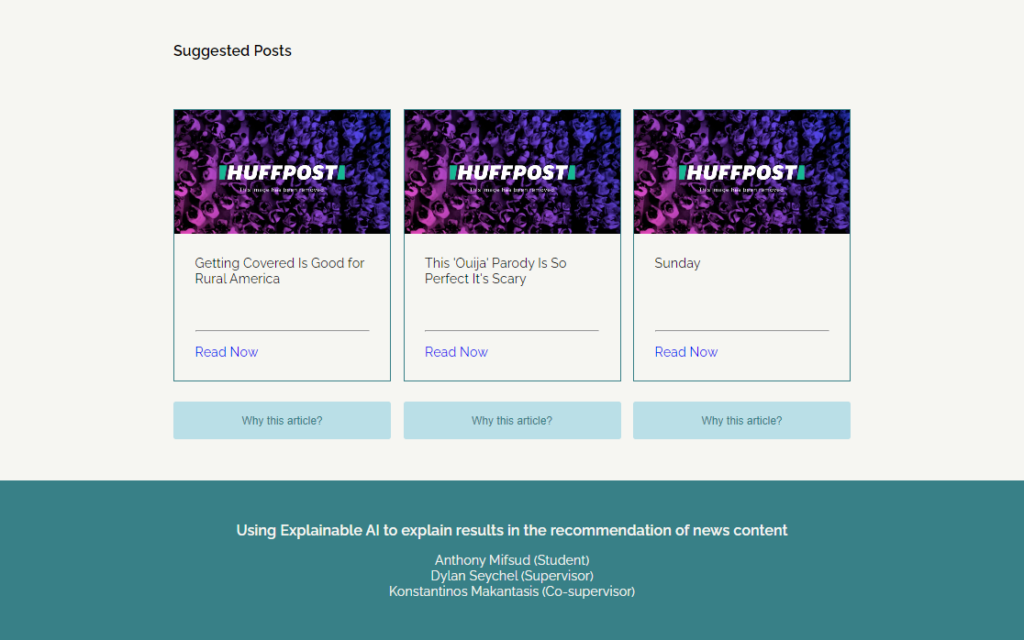
Figure 2. The recommended articles shown to the user
Student: Anthony Mifsud
Supervisor : Dr Dylan Seychell