For years, Artificial Intelligence could have been used to help surgeons and radiologists identify breast cancer earlier and with more accuracy – yet the software hasn’t been met with enthusiasm. MICHELE La FERLA has used his Master of Science in AI to ask why and come up with a possible solution.
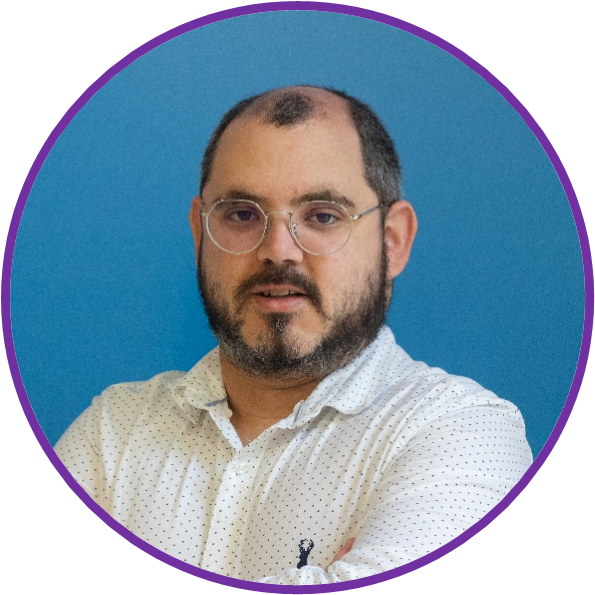
Around 300 women in Malta are diagnosed with breast cancer each year. For some, the condition may be caught too late, leading to much lower rates of treatability and even lower rates of survivability. Nevertheless, numerous studies on how deep machine learning (machines learning unsupervised) could be used to solve this have produced many positive results – but it’s not all so clear cut.
One of the issues with such software is that it’s been focused on MRIs, which are normally done well after a patient has reported a problem. This means that by the time an MRI is requested by the doctor and the results are in, certain types of breast cancer could well have spread, leading to other complications.
“This has long been understood and, in 2017 and 2018, a number of studies started looking at creating artificially intelligent software that could read mammograms, which come way earlier in the diagnostic process than MRIs do. One such study came from Google DeepMind, whose model proved to be 99 per cent accurate at diagnosing whether a person had a benign tumour [needs monitoring], a malignant tumour [needs surgery and/or chemotherapy], or showed no signs of cancer.”
Michele enrolled for his Master’s right after these papers were published and he assumed that with such high proven rates of accuracy, hospitals would be quick in implementing such technology. Yet this wasn’t happening, which is why his thesis sought to do two things: understand why radiologists and surgeons weren’t using this technology and figure out how he could change their minds.
“From data compiled through a questionnaire sent out to radiographers and surgeons who specialise in breast cancer, it quickly became apparent that the main problem was that this software couldn’t explain why it was issuing the result it was. Trust, in other words, was a very important factor in getting these key players to use it.”
To counteract this, Michele used the Deep Taylor LRP (Layer Wise Propagation) method to reverse engineer the mammogram-reading software. This, in turn, would not just tell doctors that a certain mammogram showed signs of a benign or malignant tumour (or lack thereof), but it would also show which areas of the breast X-ray were responsible for its conclusion.
“By using such software, the time a radiologist or a surgeon spends reading a mammogram can be slashed down to 10 minutes from 45. Indeed, this software is not there to replace the doctors, but to give them even more tools to make accurate and timely diagnoses, reduce human error, and even find tumours which are not that visible to the naked eye.”
It would seem that this is the end of the project and that doctors working in this field will quickly take this on, but as Michele explains, there are still numerous hurdles that need to be overcome. For a start, there are no legal frameworks – both at a national and EU level – to protect medical practitioners in the rare occurrence that the software gives an unchecked false negative or false positive result.
Moreover, such software requires vast amounts of data to learn what it needs to look for, and while currently it is being fed information from 6,000 publicly available mammograms, few patients are willing to donate their mammogram results to scientific research. This means that the algorithm cannot continue to improve, thus making the chances of mistakes or omissions higher.
To add insult to injury, a recent study has alleged that Deep Taylor LRP may not be as good at explaining results as once thought. Although, it must be said that the study has not yet been peer-reviewed and the results are from lung cancer diagnoses, which is a completely different kind of tumour.
“The human body is extremely complex and we can never take a one-size-fits-all approach,” Michele adds. “Even breast cancer can come in a variety of shapes and forms, which is why we need as many mammograms as possible for the algorithm to be finetuned to the point where even the rarest forms of breast tumours can be caught easily and in time.”
What makes such research worth fighting for is that, coupled with the doctors’ experience and expertise, it could potentially help breast cancer patients get faster diagnoses, which would lead to faster treatment and better chances of survival. The question is, are we ready to give it a chance?
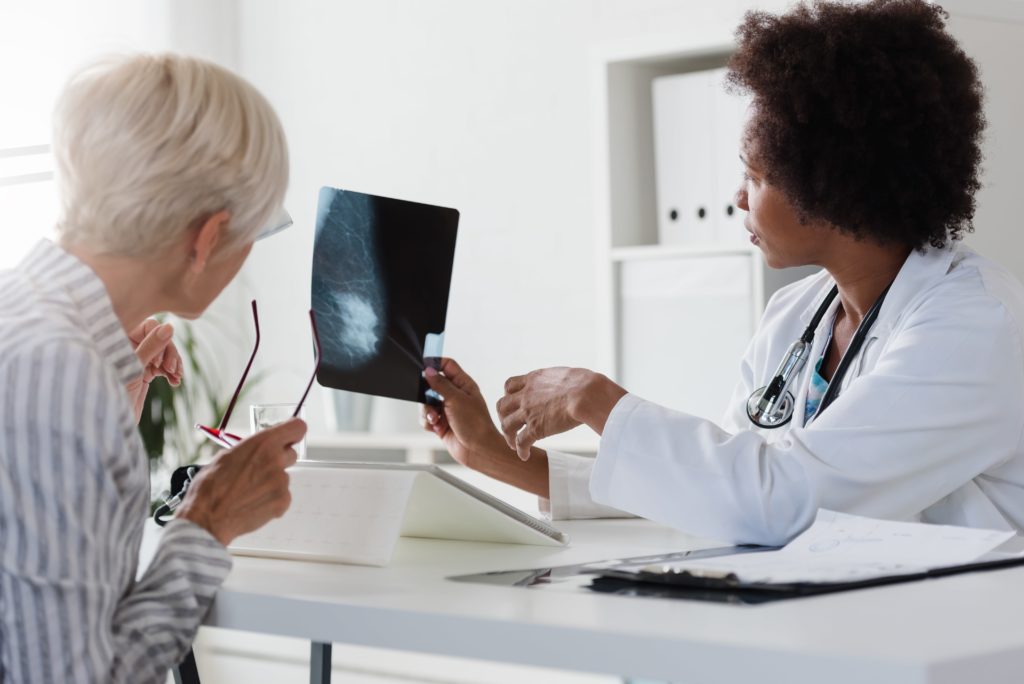