Forecasting the weather has long been point of focus among researchers seeking to achieve predictions to the highest possible levels of accuracy. The main challenge lies within the chaotic variation of temperature, where traditional techniques are unable to account for the non-linear relationships of meteorological parameters or typically require supercomputer scale-processing power to model them.
In recent years, advancements in the domain of artificial intelligence (AI) have brought to light several new tools. Through novel time-series modelling techniques, researchers could improve meteorological forecasting systems. AI-based tools have proven to be a lightweight and accurate solution for forecasting meteorological parameters. Some interesting applications include: forecasting wind, precipitation, solar radiation, sea level, and even pollutants such as PM2.5.
This research investigates the sustainability of data-driven techniques for short-term air temperature forecasting. Twenty-six months of real-world observation data from the local Marsaxlokk real-time weather station was used, spanning from July 2016 to September 2018.
An evaluation was carried out to explore the limits of the long short- term memory (LSTM) and temporal convolutional network (TCN) models when applied to the forecasting of local weather data. Each model was trained with look-backs of 12, 24, 48 and 168 hours, as well as forecast horizons of 3, 12, 24 and 48 hours. These parameters were tested in a grid search, along with model hyperparameters such as hidden layers, units per layer, dilations and kernel sizes. This
allowed each parameter to be tested multiple times, across several different architectures. As a result, it was possible to keep a certain parameter static and take the mean of all other variations. This provided a very accurate representation of the parameter’s contributions.
The findings indicated that both the LSTM and TCN models outperformed baselines and statistical techniques. Moreover, the LSTM performed marginally better than the TCN. The optimal look-
back period was found to range between 24 and 48 hours, and predictions were still found to be satisfactory for 48 hours in advance. Furthermore, it would be recommended that at least 6 months of training data be used, as a sharp deterioration in forecast accuracy tended to occur with smaller training data sizes. Finally, the results of considering external meteorological parameters within the inputted data did not produce as much improvement as expected. In fact, the results indicated that the models would perform better when only presented with past temperature and time. This result could be attributed to the short-term nature of predictions, where the other
meteorological parameters could affect the temperature over a longer period of time.
On the basis of the above, it has been concluded that AI techniques could indeed be used to forecast air temperature accurately, surpassing statistical techniques and being run on a regular
workstation. In short, AI-based solutions tend to be the ideal compromise for accuracy and efficiency.
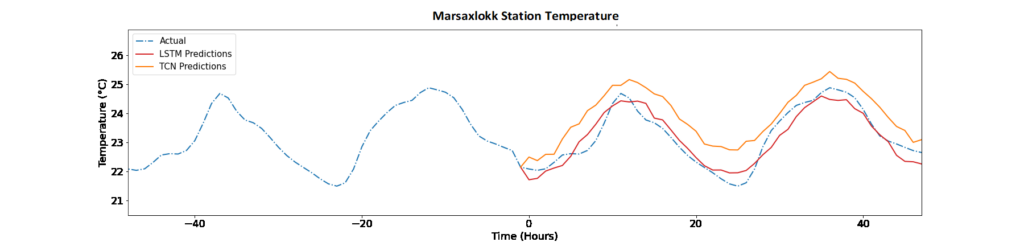
Student: Ethan Zammit
Course: B.Sc. IT (Hons.) Artificial Intelligence
Supervisor: Dr Joel Azzopardi