By enhancing the quality of CCTV images, the Deep-FIR project uses Artificial Intelligence to help criminal investigators in their work. Here, the trio of researchers behind it explain the concept.
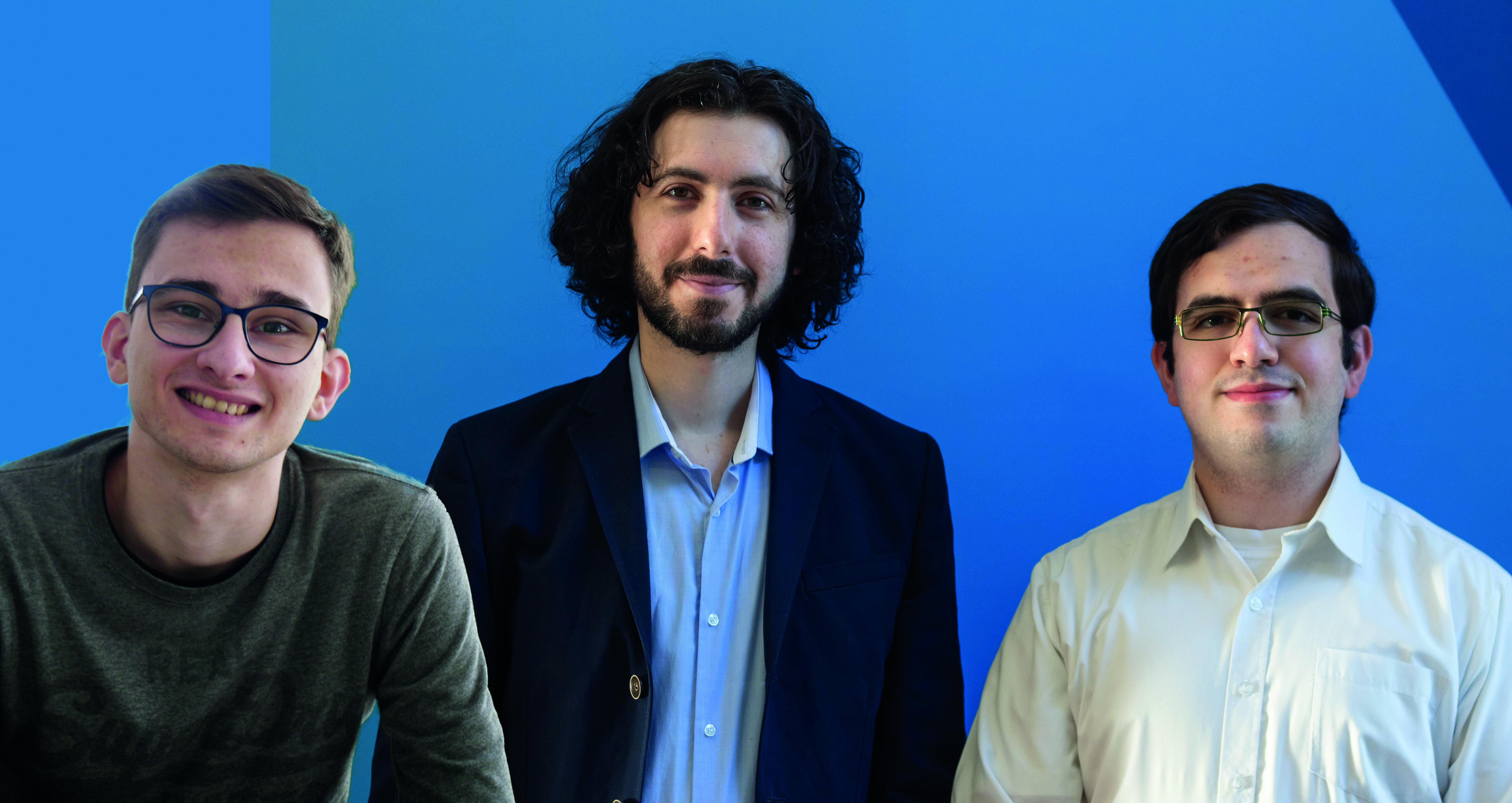
Have you seen that meme juxtaposing a crystal-clear image of the surface of Jupiter with a pixelated image from a bank’s security camera? Either way, we’re sure you’re aware that CCTV images and clips tend to lag in quality, which means they’re not always helpful to criminal investigators. However, Artificial Intelligence (AI) may be on the cusp of solving this.
“By their very nature, CCTV cameras are constantly capturing footage, resulting in vast amounts of data that need to be compressed in order to be more easily storable,” explains Dr Inġ. Christian Galea, whose PhD in Computer Vision focused on biometrics and forensics. “This often results in the reduction of image quality, which is rarely great to begin with, as such cameras tend to film in relatively low resolution and have low-quality lenses.”
This means that the low quality of such footage can sometimes defeat the purpose of why it is capturing it in the first place. After all, while CCTV cameras can help deter crime, their primary function is to aid criminal investigators in determining the identity of perpetrators.
“That may prove difficult with a pixelated image that doesn’t show much detail,” says Matthew Aquilina, a part-time research support officer currently reading for a PhD in Precision Medicine. “But this is where the Super Resolution (SR) techniques we have been working on come in.”
These SR techniques use AI models trained to do two specific jobs. The first is to make an image sharper by increasing its resolution, while the second is to help reconstruct any missing details in that image, such as by reducing blurriness.
To do this, most basic SR models use just one low-resolution image to make up the new estimate, but our trio has been seeking to create a system that could use other information, such as the gender, age, or hair colour of a subject, to improve the results further.
But this project is a sum of its parts, as each researcher has taken over the creation of a piece in the puzzle.
Keith George Ciantar, a software developer at Ascent Software with a Master’s in Signal Processing and Machine Learning, is responsible for the Meta-Attention side of the project.
“This is the process by which we can provide the AI model with supplementary information that can be used to improve the accuracy of the super-resolved image,” he explains. “So, if we know the type of camera that was used, or how the footage was compressed, we can give the AI model that information to improve image quality.”
Meanwhile, Matthew’s job is to attempt to predict any degradation an image might have suffered, such as blurring due to a poor-resolution camera or compression from trying to save space. This process, called ‘blind’ SR, allows the AI model to automatically predict and reverse such degradations.
“There are many types of blind models in the literature, and each one has its advantages and disadvantages,” Matthew asserts. “Ours has been programmed to understand how to represent each degradation so that it can then be plugged into our Meta-Attention model and boost its performance.”
While both the Meta-Attention and blind SR models are based on single images, Christian’s role uses the Face Multi-Frame SR. This means that Christian is looking to extract data from different stills in the same video to create a more complete picture.
“Although this is still in the early stages, this type of SR allows us to use information captured from multiple frames that show a subject from different angles, distances, and sharpness levels to create more accurate estimates. This is then coupled with the Meta-Attention and blind SR models to provide the clearest picture possible,” Christian explains.
Together, these three approaches have been dubbed the Deep-FIR project, and it could prove an invaluable tool for criminal investigators using CCTV images. But there’s still more to come.
“The system can also be used to restore images of vehicle license plates and old footage”
“The system can also be used to restore images of vehicle license plates and old footage,” Keith explains. “But, over and above that, we’re trying to find ways to add attributes mentioned in eye-witness’ descriptions to enforce the image and make it even clearer. This is still in the preliminary stages, but it could be a great addition to the software.”
Where the trio will take this software remains to be seen. However, with a paper describing the Meta-Attention model published in the peer-reviewed journal IEEE Signal Processing Letters, and the open-source software already up on GitHub under a dual license, Deep-FIR looks set for a bright future.