Images and photographs are an invaluable source of knowledge and information for historians, record-keepers and researchers. Old images serve as testimony to a moment in time. Sadly, however, many photographs taken in the early 20th century are at risk of being lost, as physical photographs have a lifespan of between 75 and 100 years.
Using classical image restoration techniques along with machine learning algorithms, these important images could be restored by eliminating the various defects or noise present in old photographs. The issues that could be improved on and fixed through these algorithms vary from the low resolution and comparatively lacking technology in cameras, to deterioration with time, even when kept in a suitable environment. Noise, blurriness, faded colour and sepia effect are all defects that arise from the above-mentioned factors.
Various researchers have been seeking to create an application that could solve the complex degradation problem of old photographs. The current state-of-the-art methods use different algorithms, with some being more suitable than others for different photograph and image types. Hence, this study focused on comparing and contrasting the different methods available, while also seeking to identify the weak points of current methods, and how those issue could be resolved.
It is to be noted that the classification methods used to quantitatively measure how well these algorithms perform raises an important issue where different methods use different classification approaches, thus increasing the difficulty in comparing them for performance and quality. Some of the most common evaluation methods tend to measure the ratio of signal to noise, meaning that methods that opt to focus on optimising these metrics still do not correlate to higher (perceived) image quality.
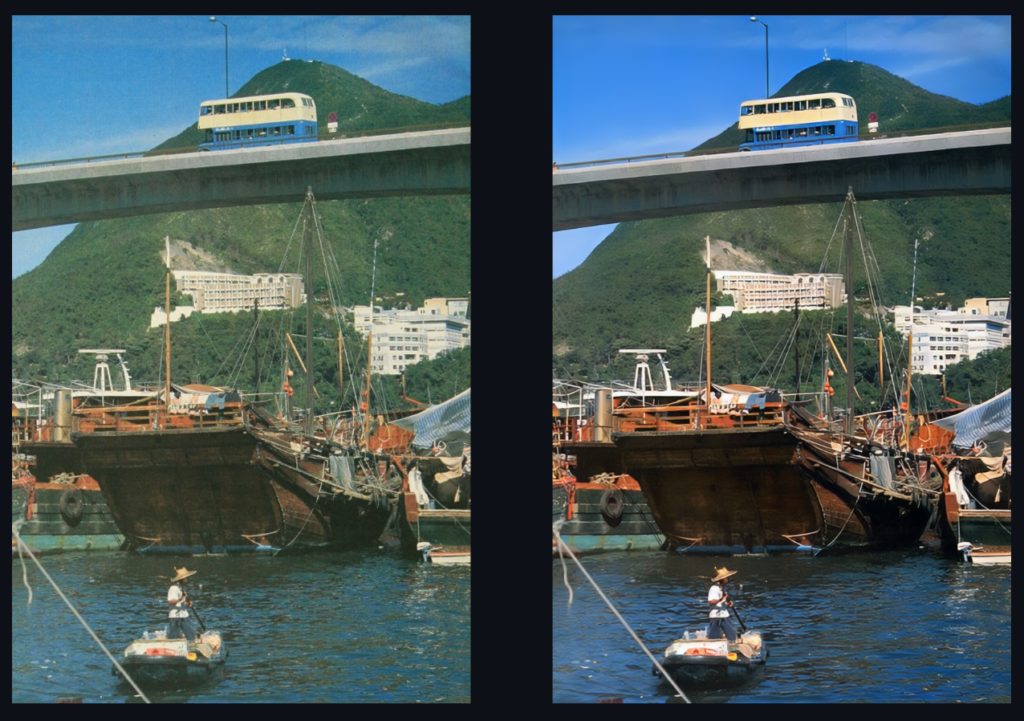
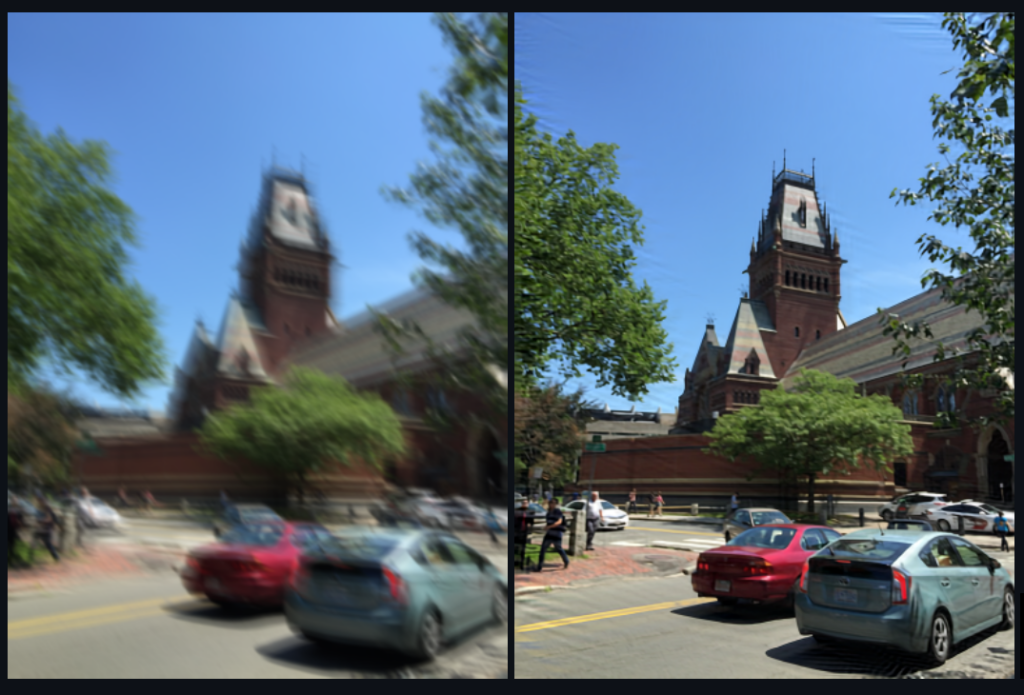
Student: Jacques Leon Patiniott
Course: B.Sc. IT (Hons.) Software Development
Supervisor: Mr Tony Spiteri Staines
Co-supervisor: Dr Clyde Meli