With more of us being put on multiple medications, the risks of drug-to-drug interactions are increasing. And while testing for these is possible, current methods are expensive and time-consuming. Now, Master’s in AI student LIZZY FARRUGIA may have taken the first step towards solving that.
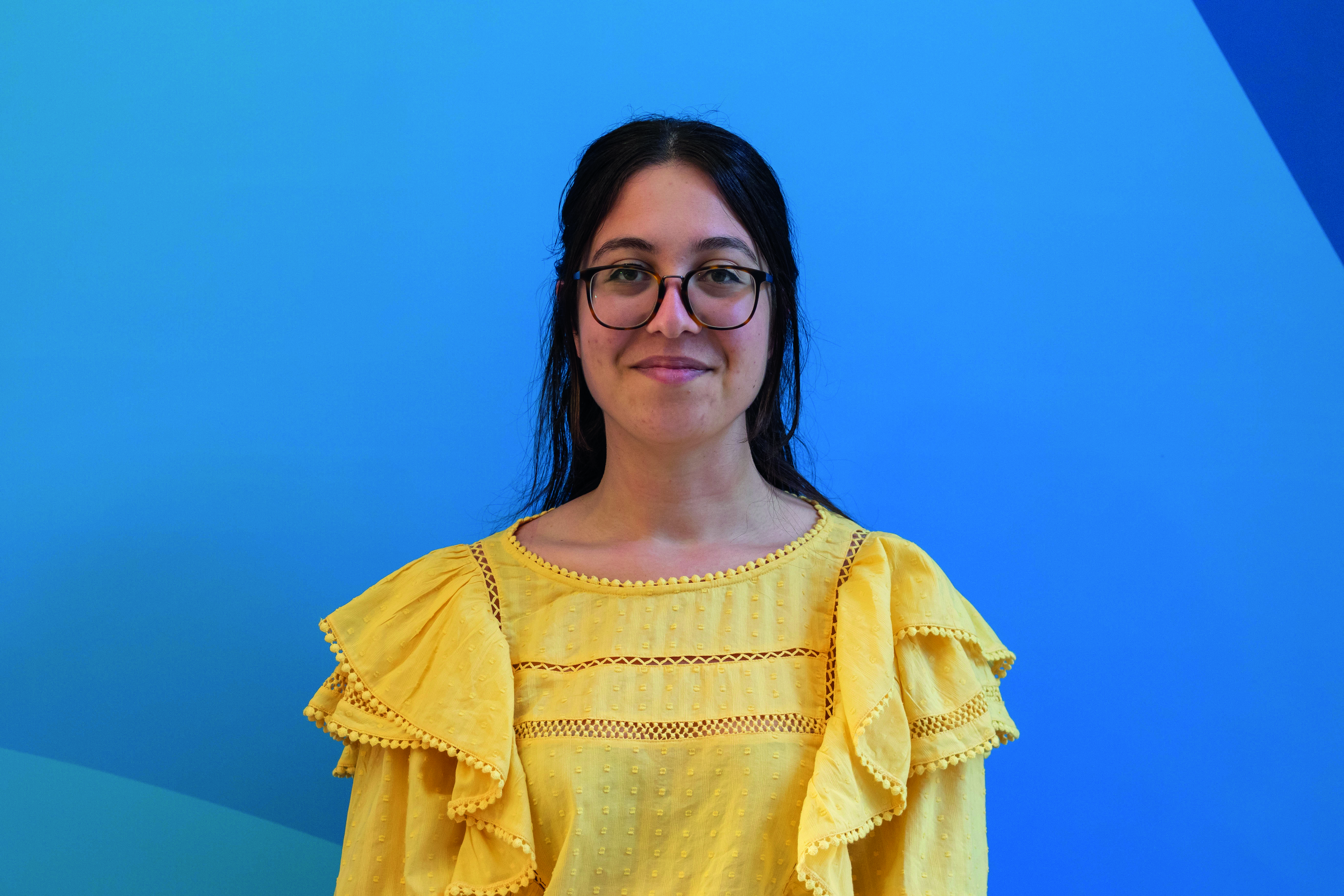
We’re fortunate to live in an age where medication can treat and prevent most diseases. However, this blessing doesn’t come without its troubles, including that consuming multiple drugs simultaneously can lead to adverse effects. Scientists are constantly testing for these, but the processes aren’t always straightforward or economical. Lizzy Farrugia’s project shows that that doesn’t have to be the case.
“The medical world knows and understands how most drugs react to each other,” she says. “In fact, pharmacists in Malta consult the British National Formulary, a highly-respected reference book that offers advice on prescribing and pairing drugs to avoid negative drug-to-drug interactions [DDI].”
Nevertheless, the way two or more drugs react depends on multiple factors. This includes their chemical makeup, what they’re meant to treat, which organs they affect, the patient’s health, and how they are consumed (swallowed, injected, absorbed, etc.)
“This means that every drug can react differently when combined with others under specific circumstances. So as more people are placed on multiple medications to treat separate issues, the number of unknown DDIs is ever-growing.”
According to a recent Polypharmacy Management Report funded by the European Union, 5% of all hospitalisations in Europe happen due to negative DDIs. That’s 430,000 people a year.
“Since the pharmaceutical industry approves hundreds of new drugs per annum, these figures could grow,” Lizzy continues. “While rigorously tested and individually safe to prescribe, it is currently tough to check how each new drug would react with every other drug under every possible condition.”
Of course, that doesn’t mean that the industry and researchers don’t do it. Scientists currently conduct in vivo (inside a living body) and in vitro (such as in a test tube) experiments, which have picked up hundreds of thousands of potential DDIs, saving countless lives. Yet, as Lizzy points out, these are costly, labour-intensive solutions.
“The other alternative is using a state-of-the-art DeepDDI, which is considered the best for researchers in this field. This is a super-powerful, Artificially Intelligent [AI] model that can predict the way drugs will react with each other using their SMILES notations.”
Here, SMILES stands for ‘Simplified Molecular-Input Line Entry System’, and it’s essentially a string of symbols explaining a drug’s molecular formula to a computer. For example, in the case of aspirin, the SMILES notation is ‘O=C(C)Oc1ccccc1C(=O)O’.
“This, however, comes with its limitations. We don’t have such notations for all drug types just yet, and even when they’re available, they sometimes over-simplify the structure, leading to incomplete results.”
During her research, Lizzy came across another method that could be used: the knowledge graph (KG), a semantic network that shows the relationship between two or more entities. This, as she explains, had been used to find unknown DDIs in the past with promising results, which is why she decided to follow this path.
“To build the KG, I first had to create an inventory of pertinent data, which I got from reliable sources like the DrugBank [a database of known drug interactions], the Bio2RDF [a database of the studies of living organisms], and the ATC Classification [a drug classification system managed by the WHO].
“Then I mapped the relationships between the different points in the data, showing the system what was and wasn’t connected. Finally, I tested the KG’s validity to confirm the system would work in a real-life scenario.”
With this phase completed successfully, Lizzy moved on to the prediction phase, for which she connected the KG with a deep neural network. This is a stacked system with each layer observing and analysing data similarly to how the human brain does.
“A cheaper, less labour-intensive, and potentially more accurate alternative”
“Its first task was to predict known DDIs so I could make sure it worked. This achieved an F1 score of 91.94%. This is fantastic and means we’re on the right track to creating a system that could offer a cheaper, less labour-intensive, and potentially more accurate alternative to help predict DDIs and, maybe, even suggest safer drug combinations in the future,” Lizzy concludes.
Although the work on this system isn’t complete yet, it’s already extremely promising. So, who knows? In the future, it may end up saving someone’s life.