The sea between Malta and Sicily is constantly monitored to ensure our maritime security and avert oil spill disasters, yet the data we collect can sometimes be incomplete. Now, Master’s in AI-graduate ANNE-MARIE CAMILLERI may have figured out how to fill those gaps.
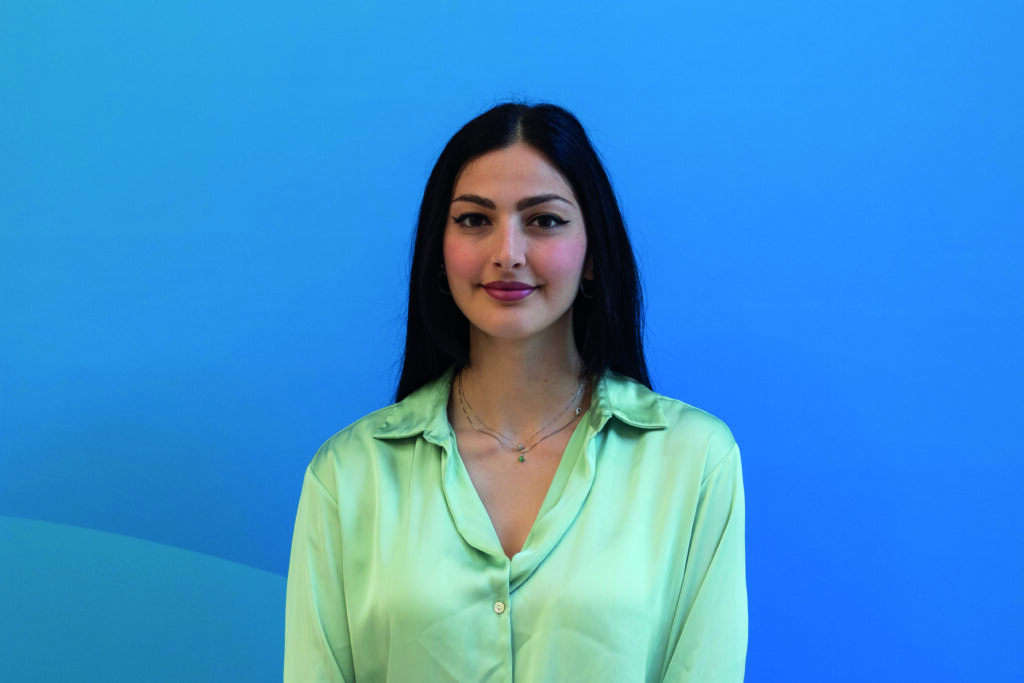
Whether we’re talking about aviation, architecture, or security, our modern way of life depends on the collection and analysis of data. But what happens when the technology that provides this information breaks down or suffers connection losses? The answer is data gaps, which could have severe consequences for the task at hand. Thankfully, Anne-Marie Camilleri has been working on a system that could help us fill the gaps for the four High-Frequency Radars (HFRs) that monitor a particularly pertinent area of our seas.
“HFRs are antennas that bounce high-frequency radio waves off the sea’s surface, hence why they are always located at the water’s edge,” Anne-Marie explains. “These help scientists collect oceanographic data, which is crucial for both researchers and Malta’s authorities.”
There are currently four such HFRs monitoring the stretch of sea that separates the Maltese Archipelago from the southernmost tip of Sicily, known as the Malta Channel. These are located at different points in Malta, Gozo, and Sicily, and at least two of their frequencies must overlap for data to be collected.
“This overlapping provides the University of Malta and other relevant authorities with the vector data [longitudes and latitudes] required to monitor the seabed, be alert for potential oil spillages, and maintain our maritime security.”
Collecting and analysing this information is therefore essential. Nevertheless, as Anne-Marie points out, technology operating on the same radio frequency as the HFRs can sometimes interfere with the signal. Meanwhile, extreme weather events could result in a complete system breakdown.
“It’s very fortunate that Artificial intelligence can help us … fill these gaps”
“When this happens, the information we receive is incomplete, resulting in data gaps. This can reduce our maritime security or even cause delays in identifying environmental disasters. So it’s very fortunate that Artificial intelligence (AI) can help us avoid this.”
Anne-Marie employed machine learning techniques to fill these gaps, a process through which a computer can be taught how to think like a human being. The exercise began with Anne-Marie gathering the data collected by the four HFRs over the previous two years. A computer then analysed this data set to extract knowledge and identify patterns, allowing it to fill gaps in the data even when the HFR signal is down.
To achieve the best possible outcome in this operation, Anne-Marie ran the data set through three different machine learning models before comparing their results. The Long Short-Term Memory Neural Network (LSTM) emerged as the winner.
“This neural network, which mimics how our brains work, can remember select patterns for longer, making it ideal for our task,” she explains. “But what’s even better is that we now have a deeper understanding of how to fill such gaps and make predictions.
“For example, we now know that including wind data from satellites in the mix doesn’t significantly improve results. We also know that older data is relevant to training a system, meaning it doesn’t need to be retrained with new datasets after set intervals. And, finally, we know that the data from the six hours immediately preceding a gap is the most important for the computer to fill a gap.”
Yet this system goes a step further. Through its knowledge, it can forecast future data up to 24 hours in advance, allowing researchers and authorities to anticipate certain events, such as strong currents before they even occur. And this opens up a whole other world of possibilities.
“I’m thrilled with the work so far, and I’d like to publish my findings in the future. But there’s much work to be done before that, and I would be happy if a prospective student took it over,” she concludes.
What will happen next with Anne-Marie’s project remains to be seen. Nevertheless, it cannot be denied that this is an excellent example of a system with transferable skills: all it needs is a different data set, and it can predict events in other countries and other seas. That in itself is a promising development that could guarantee its future.