Over recent years, technology in nature studies offered assistance in academic research and solutions for environmental crises. Machine learning is an approach that could continue to further the technological progress of this field. However, it is also widely acknowledged that machine learning techniques require a considerable amount of data, which could be particularly challenging to compile. Maltese botany is one area of interest that lacks the required quantity of data, resulting in limited technological advancements in the said field. Training a deep-learning network with insufficient data would cause overfitting. Therefore, auxiliary techniques would be required in order to overcome this issue.
The first part of this study investigates the training of a deep learning model that makes use of a limited training dataset utilising techniques such as data augmentation, data scraping and transfer learning. The deep learning model that was considered consisted of 50 categories, incorporating species endemic or indigenous to the Maltese Islands (such as the Maltese sow thistle). Data scraping was used for the collection of all available data. This technique did not generate sufficient training data, with sample sizes ranging between 10 and 217 for each category. Subsequently, data augmentation was used to enhance the dataset with augmented data. It was concluded that data augmentation performed well on both the training data and the testing data, generating a higher accuracy model than when data augmentation was performed solely on the training dataset. Different transfer learning models were also evaluated, and it was observed that the VGG-16 model outperformed the other models considered, generating an accuracy of 47.87%. This indicated that, although techniques such as transfer learning and data augmentation were implemented, the accuracy of the model was still relatively low.
While supporting the training of the deep learning model, these techniques were not a replacement to the data. This low accuracy of an improved off- the-shelf model showed the relevance of the initial hypothesis that citizen science (CS) would be required to enhance deep learning models. Consequently, this study has experimented with CS as a data-augmentation technique. CS and mobile-communication technology enable volunteering participants to contribute to the crowdsourcing of data. Since CS depends on the structure of society and culture, a questionnaire-based survey was conducted to determine the opinion of the general public regarding level of interest and willingness to participate. Out of the 243 respondents, it was only 13.2% who stated that they were not interested in such a mobile-communication system for crowdsourcing data. Due to the existing COVID-19 situation, the application could not be distributed and tested by the general public. However, the 230 images that had been gathered previously were used as an evaluation of the communication system. The collected data was also utilised to generate visualisations of the current distribution of Maltese flora.
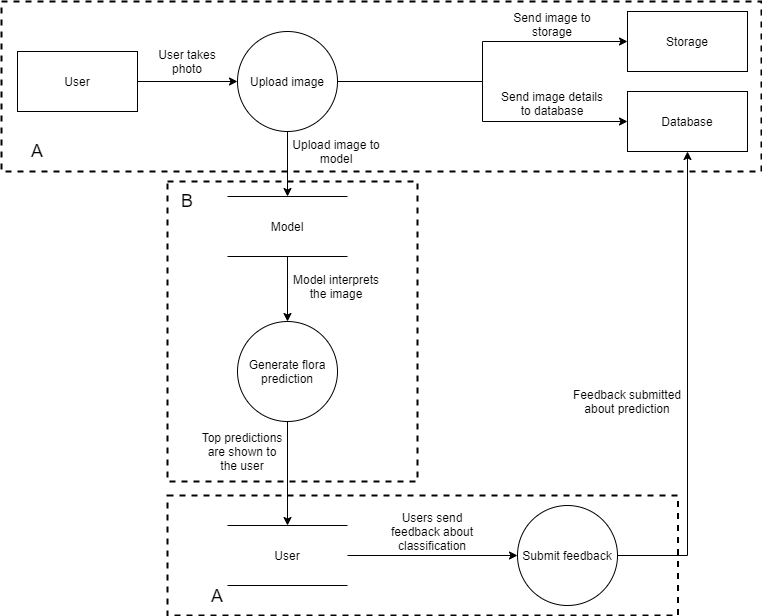
Drawing from the research results, as outlined above, it was concluded that the use of CS would be essential for the improvement of deep learning models, when required to be employed in more widespread applications.
Student: Chantelle Saliba
Course: B.Sc. IT (Hons.) Artificial Intelligence
Supervisor: Mr. Dylan Seychell
Co-supervisor: Prof. Joseph Buhagiar