In light of the recent progress in machine learning (ML) and artificial intelligence (AI), researchers aim to apply techniques for improving and automating certain facets of clinical practice. One of the more intriguing and compelling applications of modern computing in a healthcare context is the early detection and prediction of disease. In the case of epilepsy, the prediction of seizure onsets would allow patients to adequately prepare for such recurrent episodes of convulsion, thus improving their quality of life. Although seizures are preventable by specific medication and therapies, it is not uncommon for patients to suffer from intractable seizures caused by drug-resistant epilepsy. The prediction of seizure onsets would allow patients some relief in knowing when to be prepared and when to avoid activities that could be potentially dangerous, such as driving.
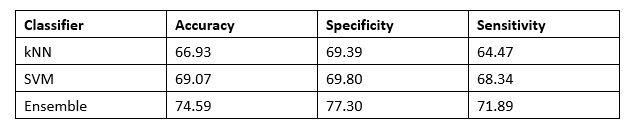
This dissertation presents a review of the performance of a set of supervised machine learning methods for the task of seizure prediction. The study involves using a dataset that includes non-invasive scalp electroencephalography (EEG) signals, which are brain electrophysiological readings that do not involve surgery. The findings are presented and compared with current research in the field. Upon completing data labelling and pre-processing, statistical and wavelet features from the signals were subsequently extracted, which in turn was used for training the machine learning models. The results obtained from the k-nearest neighbour (kNN), support vector machines (SVM) and ensemble classifier were compared accordingly. The results have been reported on the CHB-MIT dataset, which includes 198 seizure readings from 22 patients suffering from intractable seizures. The study suggests that the three above-mentioned methods deliver a similar level of efficiency. However, the ensemble classifier achieved a higher specificity, sensitivity and accuracy.
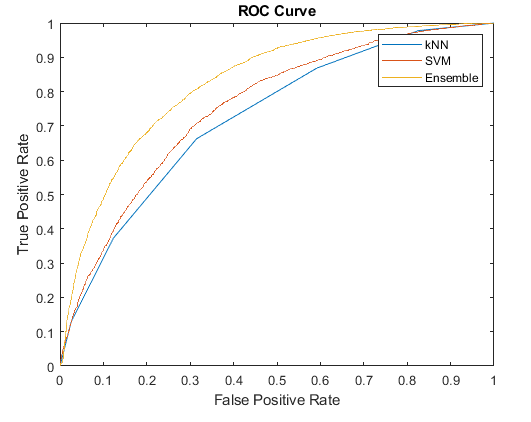
References/Bibliography:
[1] Shoeb, Ali & Guttag, John. (2010). Application of Machine Learning To Epileptic Seizure Detection. ICML 2010 – Proceedings, 27th International Conference on Machine Learning. 975- 982.
[3] (4 September, 2017). Epilepsy – Treatment – NHS. Available: h ttps://www.nhs.uk/conditions/ e pilepsy/treatment/.
Student: Jonathan Cauchi
Course: B.Sc. IT (Hons.) Computing Science
Supervisor: Dr. Lalit Garg