Gait analysis is the systematic study of walking patterns. Typically, expensive and intrusive marker- based methods, in conjunction with multiple infrared cameras, are used to produce kinematic data that concisely quantifies the walking behaviour of a person. Subsequently, specialists interpret conclusions from the kinematic data to make diagnoses. The aim of this research is to develop an automated alternative to marker-based methods for gait analysis.
Figure 1. Pose estimation of a video frame with side and front views of the participant. Person detection is followed by keypoint detection resulting in pose-estimation graphs
The study proposes an automated method based on artificial intelligence that is able to achieve kinematic data consisting of varying left and right joint angles for hips and knees. All of this was obtained from videos capturing the side and front views of a walking subject. The method makes use of pose estimation, as well as a pipeline of techniques for calculating and processing kinematics. Included in the pipeline is a procedure based on ankle displacement for sampling walks into cyclic periods known as gait cycles.
Video data for the automated method, as well as marker data for a well-established marker-based method, was collected simultaneously in a biomechanics lab for comparison. Per participant, the automated method achieved a minimal error of about 3.14 degrees for left and right joint angles of hips and knees. This coincides with the measurement error of marker-based methods, which most studies have found to be less than 5 degrees (McGinley et al., 2009).
A binary classification experiment was performed with the kinematics of the automated method, in samples of gait cycles. Support vector machines achieved a 79% accuracy in detecting whether the walk of a person was normal or abnormal. The automated method has proven to be more suitable for data collection and processing than marker-based methods, and it may be utilised for further gait-classification procedures. It reduces the effort and financial investment needed for gait analysis, leading to a wider diffusion in the healthcare and research communities. The proposed automated method could also serve as a basis to train a classifier with sufficient kinematic data to accurately deduce gait-related conditions, and minimise the involvement of specialists for diagnoses.
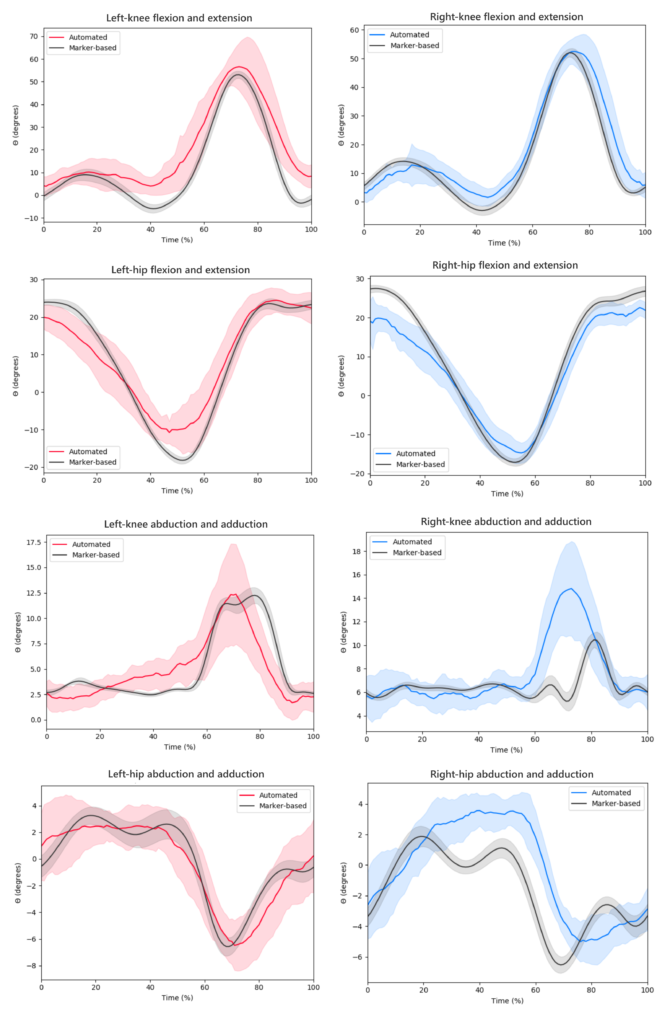
References/Bibliography:
[1] Jennifer L. McGinley, Richard Baker, Rory Wolfe, Meg E. Morris, The reliability of three-dimensional kinematic gait measurements: A systematic review, Gait & Posture, Volume 29, Issue 3, 2009, Pages 360-369
Student: Russell Sammut Bonnici
Course: B.Sc. IT (Hons.) Artificial Intelligence
Supervisor: Prof. Alexiei Dingli
Co-supevisor: Mr. Dylan Seychell