Image understanding (IU) is a subfield of computer vision (CV), which seeks to detect the semantics of an image. Object detection in images is the first step in IU. However, a list of objects alone is not enough for most applications in a number of areas, such as robotics, image-description generation and visual question- answering. Previous work dedicates significant effort in the detection of attributes (e.g. green box) and relations (e.g. on top of). On the other hand, comparatives (e.g. larger or taller than) is a relatively unexplored area. In this project In the absence of an existing dataset, this project required the building of data-driven pattern recognition (machine learning) models are built to detect comparatives in images. Moreover, a literature survey on gradables and comparatives was carried out before collating a suitable dataset.
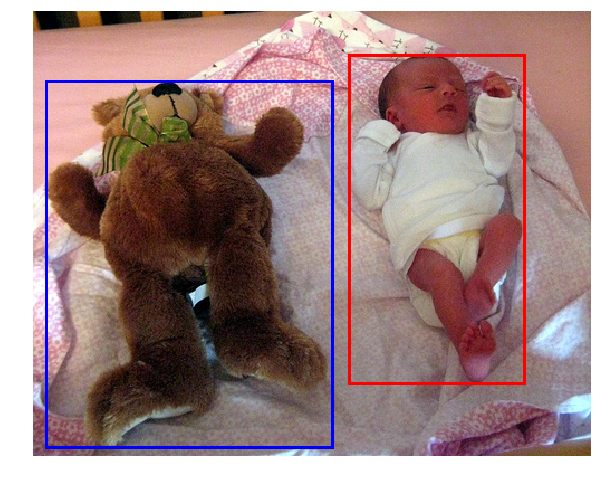
In the absence of an existing dedicated dataset, one was collated from readily available, human-annotated, easily obtained datasets, more specifically and primarily from: single-label annotation datasets; image-description datasets and visual question-answering datasets. The dataset consists of images and identified object pairs with respective bounding boxes, and the corresponding relevant comparative, used as a gold label for the models to be built. This could be seen in Figure 1, where the comparative ‘bigger than’ was assigned to the object pair (‘teddy bear’, ‘person’), and the objects identified with bounding boxes (blue and red respectively). The models make use of language features ‒ as well as geometric features computed from the selected bounding boxes – in order to predict the comparatives relating to the identified pair of objects. The models developed were analysed and compared.
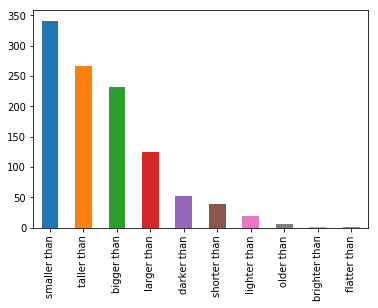
This study also discusses the challenges that arise in the tasks that attempt to extract semantic value from images using a set of features, as well as the challenges due to the ambiguity of the comparatives from a language perspective. The list and distribution of comparatives from the collated dataset can be seen in Figure 2.
The visual comparatives dataset and the results and critique of the models developed for the purpose, set the foundations for future work in this area.