Our planet has experienced numerous environmental events throughout its history, and there has been significant interest over the centuries to the present day to discover more about them when they happen.
Each event could be said to either be the result of abnormal meteorological behaviour, or cause further meteorological changes. A wildfire, for example, will always contribute to an increase in temperature, due to the fires involved. A flooding would most likely be caused by a substantial increase in rain that happened in the vicinity of the area.
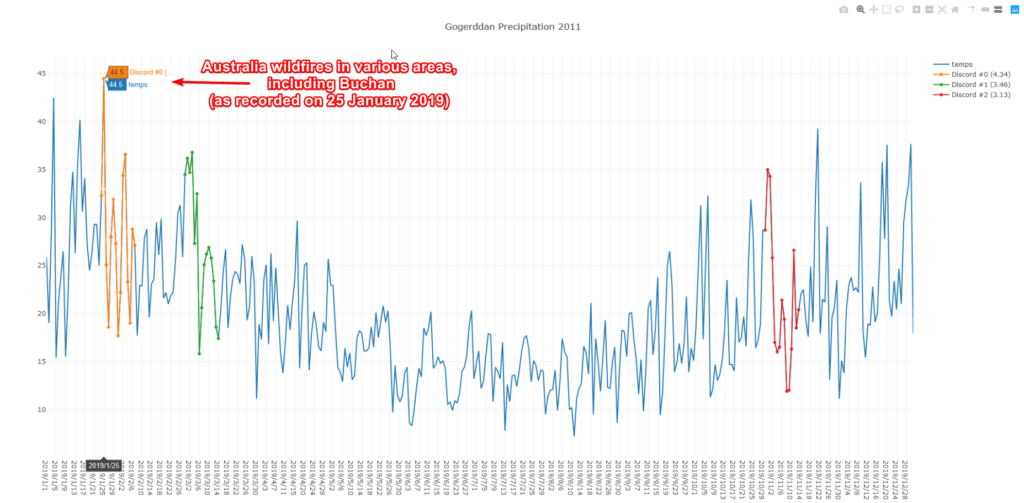
Most events have a primary cause or direct effect that could be detected, if sought or investigated. A drought is usually the result of a significant lack of rainfall over a long period of time, and so on.
The system being proposed in this study utilises time series data in order to detect anomalies, which are subsequently presumed to correlate to a specific event. It processes a dataset for the primary datapoint within it (temperature, precipitation, etc.), and then executes a discord-discovery algorithm over it in order to identify any anomalies. Once this is done, the discords could then be linked to a news article by utilising the time span of the anomaly, and some additional parameters, such as which query to submit. A group of articles is then requested using the application programming interface (API) of the relevant news source, the most relevant ones being those revolving around the event that occurred. For example, one could input year-long precipitation data for a certain city in the UK, enter ‘UK flooding’ as information request, select The Guardian as news source, to be presented with the available articles regarding a flooding in the city under review.
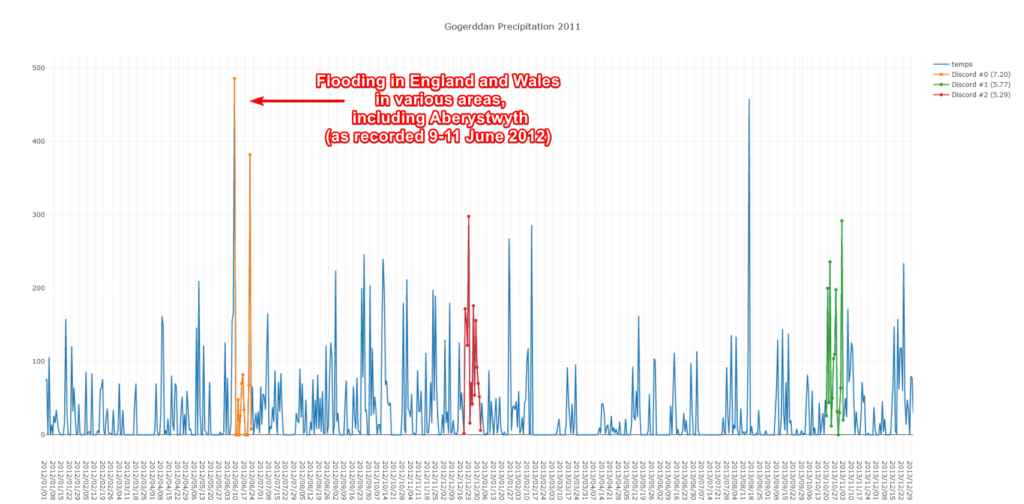
Although the current system is a prototype, it could easily be extended. One could implement APIs for further news sources, or attach the dataset to the system itself, in order that it could dynamically find the data and even generate the query itself, requiring even less input from the user. Observing the results obtained on temperatures linked to wildfires, and precipitation for instances of flooding, the system detected such events with very high accuracy, confirming that the significant anomalies in the time series data strongly suggest the occurrence of an associated event.
Student: Andre Jenkins
Course: B.Sc. IT (Hons.) Artificial Intelligence
Supervisor: Dr. Joel Azzopardi