This study investigated the potential improvement in word-prediction performance by enriching language models with contextual data, such as image classification and speech recognition. A deeper understanding of context could have significant implications in the field of natural language processing (NLP), leading to more accurate and robust models.
The system utilised four image classification models, including VGG-16, VGG-19, and Inception V3, which were pre-trained on extensive image datasets to predict five indoor classes (bathroom, bedroom, dining room, kitchen, and living room) from a house-rooms image dataset. Google Cloud Speech-to-Text was employed to transcribe spoken words into text. Large language models were then used to evaluate the effectiveness of the image classification and speech recognition, by integrating the predicted room and the information obtained from speech transcription before the user input.
A customised dataset was created with images of rooms, recorded speech, and text input, including the desired predicted word. The data was transformed into textual information and used as input for the large language models. A separate language model generated the text input to ensure that the primary model was tested with new data.
The results demonstrated that the overall performance of the language model in word prediction improved by 15% when adding the additional contextual information. Furthermore, speech-recognition data provided the most substantial impact on the model’s accuracy.
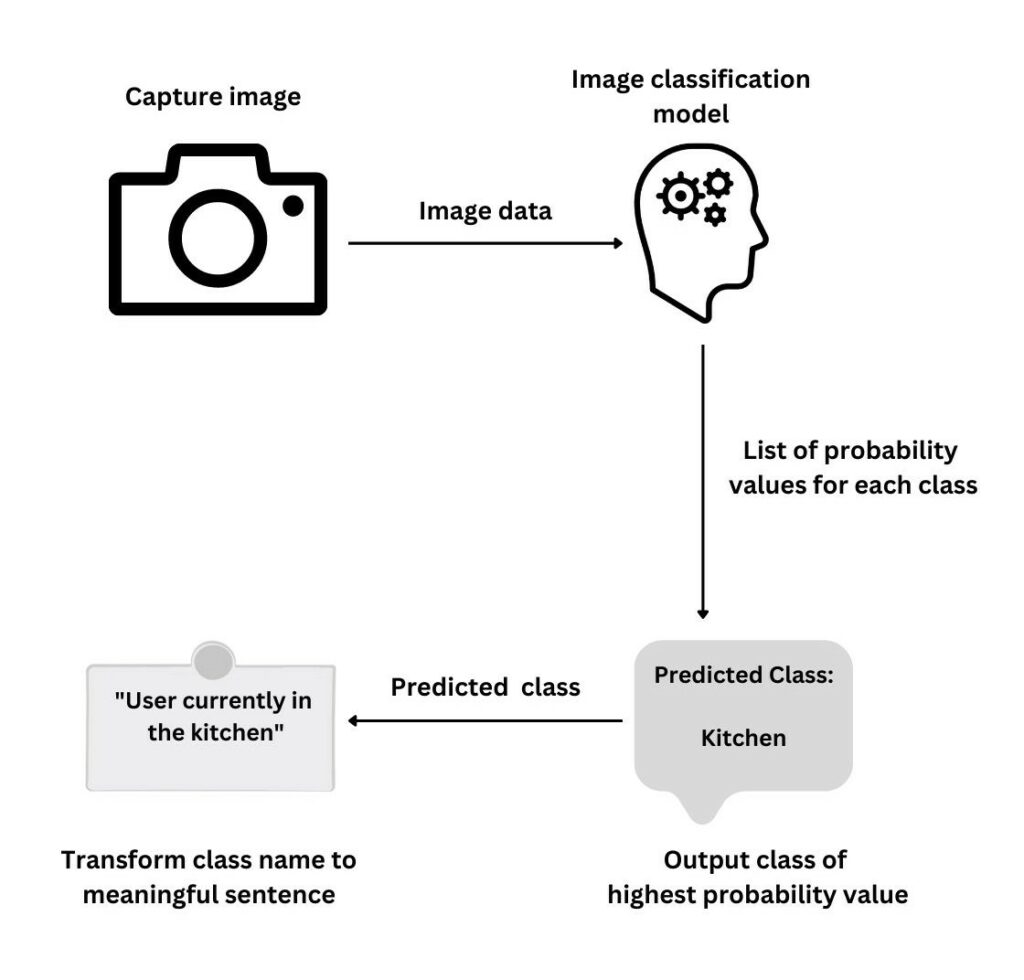
Figure 1. Image classification
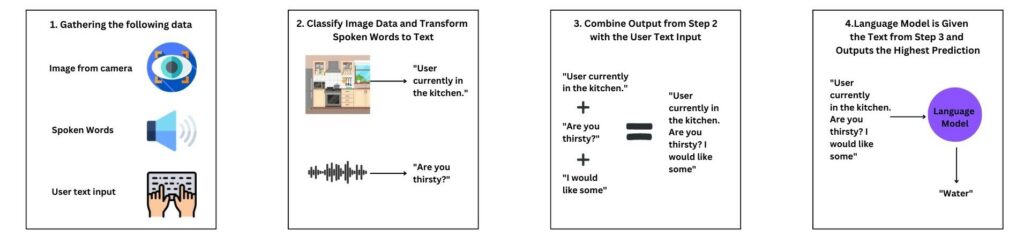
Figure 2. The proposed solution
Student: Liam Bugeja Douglas
Supervisor: Prof. Alexiei Dingli