This study investigated the feasibility of using NBA (National Basketball Association) performance statistics in injury prediction models, to extract potential risk factors in order to avoid injuries that tend to occur amongst professional basketball players, more specifically those playing in the NBA, which is the largest basketball league in the world. The investigation employed a variety of prediction models. This was done in order to assess the capabilities of performance statistics data in different types and variations of machine learning (ML) models, and the potential for their use in future, more complex prediction models.
The prediction models used for this study were ML models, which are algorithms capable of finding patterns, or making a decision or prediction on the basis of previously seen data. The prediction model is trained on past data, thus enabling it to make predictions on new, unseen data. This study made use of different ML methods to help achieve its goal, including principal component analysis (PCA), which was utilised to extract information on the performance statistics and their correlation with injuries, primarily for injury prediction models. Examples of some of the models that were used are: random forest sampling, XGBoost, and neural networks with different optimisers, such as Adam, AdaGrad and stochastic gradient descent (SGD).
Injuries, especially severe ones, have a detrimental impact on the performance and careers of affected players, and the collective performance of their team. Identifying possible injury risks and having an effective injury prediction model in place would equip organisations and coaching staff in any league with the best possible tools and knowledge to address the issues caused by the injuries.
Existing injury prediction models for basketball and NBA have mainly focused on using physiological factors, such as age and weight, as well as injury history. Although this study also made use of other factors, the main aim was to expand on the known knowledge of this area by exploring the potential use of performance statistics as the main or additional data in injury prediction models. Certain correlations between performance statistics and injuries have already been identified. For example, the accompanying graph, which has been sourced from a publication on knee injuries and associated factors in the NBA [1], charts knee injuries against minutes per game. Correlations such as those displayed in the said graph show the potential of the application of performance statistics in injury prediction models.
The data for this study was gathered from publicly available sources, namely: Basketball Reference for performance statistics, and Pro Sports Transactions Archive for injury data. Performance statistics such as points per game, assists rebound, fouls and minutes played were also used in the research.
The findings of this study have possible implications for the NBA, its stakeholders and any injury-prediction-model developers. The said findings offer pointers as to whether performance statistics could be used effectively for injury prediction models for basketball players, more specifically those playing in the NBA.
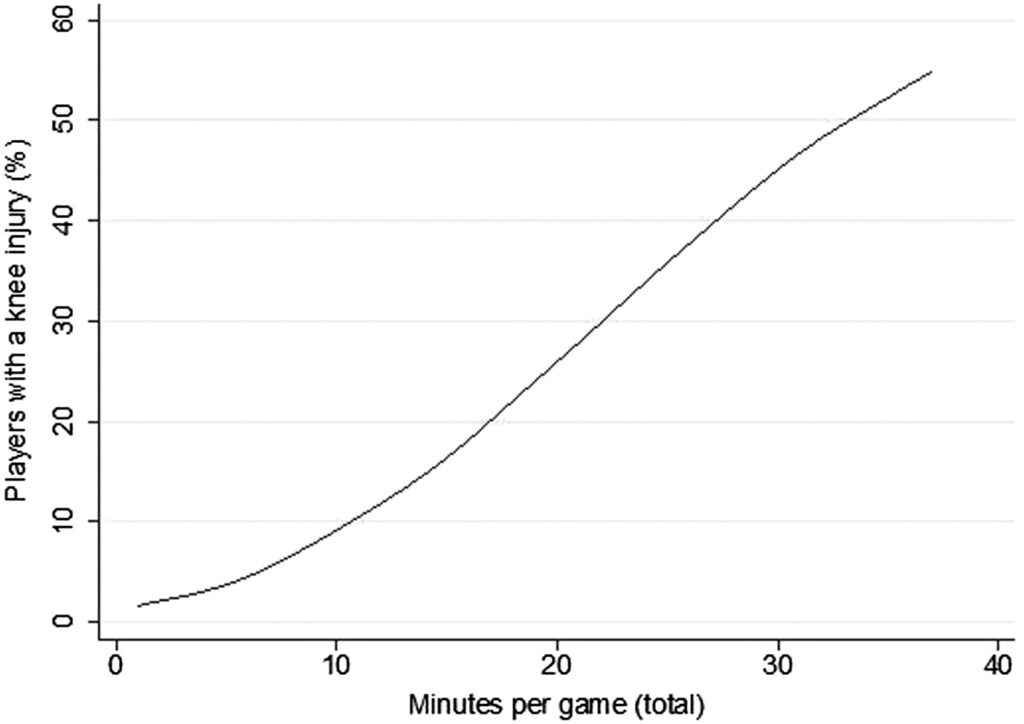
Figure 1. Graph conveying the percentage of NBA players who suffered a knee injury by minutes played per game (regular season and playoffs) as sourced from Tummala et al.
Student: Andrea Vella
Supervisor : Dr Conrad Attard