Banks are about to get a helping hand when it comes to deciding whether an applicant should be granted a loan or not thanks to LARA MARIE DEMAJO’s Master’s in Artificial Intelligence.
Any bank will tell you that one of the loan officer’s toughest jobs is deciding whether or not an applicant will be able to repay their loan as determined by their contract. With so many variables to look at, it’s not hard to see why it sometimes takes ages for a decision to be made. Yet a new credit scoring model currently being worked on by one of the students reading for a Master’s in Artificial Intelligence, Lara Marie Demajo, could very well halve the loan officer’s job, leaving them with more time to spend on what really matters.
“Using a machine learning model that is equipped with an XGBoost algorithm, I have created a credit scoring system that not only analyses the data inputted into it to come up with a confirmation or denial of an applicant’s loan, but which also gives a detailed explanation of the logic it used in making its decision,” Lara explains. “This, in my opinion, as well as in that of the bank managers I have spoken to, will result in a more seamless and fair way for technology to help loan officers in their job while cutting waiting time for applicants.”
For those who are not familiar with credit scoring, this is a system which is used by banks to decide whether a person should be given a loan or not, as well as how big that loan should be, judging by past credit, the person’s wage, and other loans and assets, among other factors. The current situation, however, sees loan officers often doing all the work themselves, even for cases where the results should be a black-andwhite affair.
“The idea here isn’t to replace the loan officer but to offer them assistance that can save them precious time on applications which are easy to determine based on the information provided. This means that the loan officers could then use their time to go over applications which are more sensitive or less straightforward.”
The explanation given by the software will also prove incredibly useful when speaking to the prospective applicants as it breaks down the reasoning behind its decision. Together, the loan officers and applicants could then discuss what changes would need to be made in order for them to get a loan.
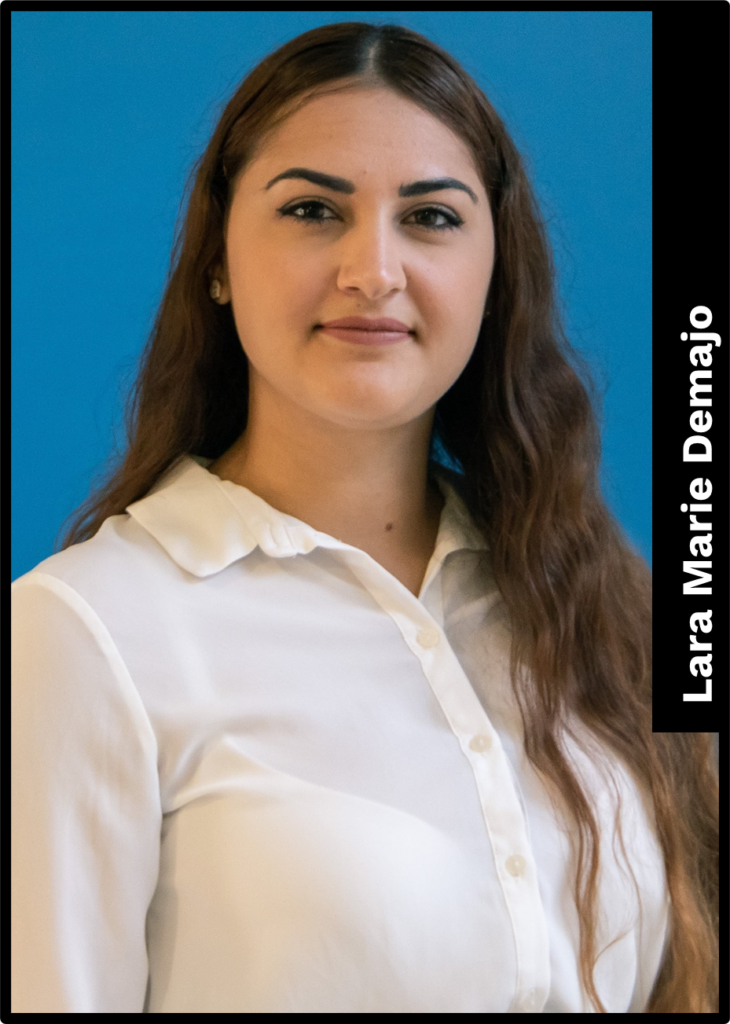
“Of course, the software does not have the final say. Instead, it goes through the information and gives the predicted outcome based on the logic it has learned from past loan applications, which can then be further analysed by the loan officer,” Lara continues. “The explanation, meanwhile, also aids banks in observing the laws related to GDPR and ECOA, which require them to share the reason behind their decisions.”
For this system to exist, Lara trained the model using large data sets containing information of different loan applications from two foreign credit institutions. Moreover, she analysed if the provided explanations are easy to understand by gathering opinionative results through interviews with seven loan officers, as well as a hundred questionnaires filled in by the general public. As a result, this state-of-the-art system is much more sophisticated than the counterparts currently used by the majority of the banks.
“Needless to say, there is room for improvement in terms of accuracy, but there may be a time when such systems could also help determine the cases which are not so straightforward. Even so, one of the most important things that have come out of my research is the fact that younger generations are more inclined than ever to trust such software with important decisions. Indeed, it shows a clear shift in mindset, and it paves the way for the world we’ll be living in in the years to come.”
In the future, Lara is adamant that more AI systems will use similar approaches not just to make choices but also to explain them. This, for example, would be particularly useful when it comes to autonomous cars, which make decisions intuitively and autonomously that affect both those in it, as well as those driving nearby, pedestrians and so forth.
“Knowing why the AI software decided that Option A was more viable than Option B helps us build a better rapport with it, as well as understand any pitfalls,” Lara adds.
Of course, not all situations can be tackled using logic on its own. How would the world fare if medical choices were based solely on logic rather than logic coupled with empathy and humanity? Yet, that doesn’t change the fact that having an independent arbitrator can help us make more accurate choices in a shorter time span.